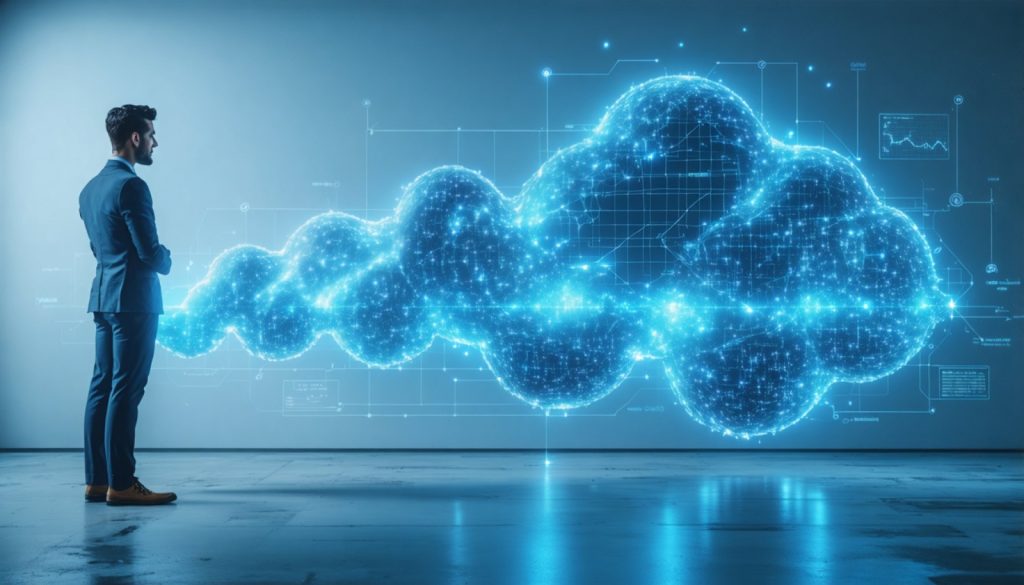
- The AI landscape faces a mix of innovation excitement and trust concerns, leading to the “trough of disillusionment.”
- Key sectors like healthcare, finance, and government remain hesitant to fully embrace AI, fearing catastrophic risks from potential glitches.
- Historical parallels with the dot-com bubble suggest caution in overvaluing AI’s potential without practical backing.
- Despite growing adoption, 80% of companies see no earnings increase, indicating challenges in human readiness, not AI tech limitations.
- Behavioral economics points to trust issues and organizational inertia as barriers to effective AI integration.
- Successful AI-human collaborations require trust, transparency, and combining AI’s pattern recognition with human creativity.
- Businesses and investors should focus on trust-centric AI frameworks, explanatory AI, and human-AI collaboration for sustainable success.
- The future of AI lies in fostering trust alongside technological innovation, fundamentally reshaping industries.
The landscape of artificial intelligence (AI) is a curious blend of cutting-edge innovation and age-old human hesitance. The initial euphoria over AI technologies has been tempered by a reinvigorated focus on trust and practical implications. Tech visionaries and industry leaders are now confronting the sobering reality of the so-called “trough of disillusionment.”
For years, tantalizing stock surges of AI behemoths like Nvidia, Palantir, and C3.ai have driven market excitement. Yet, the lingering question looms: Are we equipped to place our trust in non-human intelligence? The apprehension is palpable as pivotal sectors like healthcare, finance, and government, those ripest for AI disruption, hesitate to fully embrace these technologies. Here, a glitch isn’t a minor inconvenience; it could be catastrophic.
A backdrop of history underscores the current scenario. Take the late 1990s, when investor fervor over internet stocks soared beyond practical valuations, leading to the infamous dot-com bubble. Similarly, the promise of AI, echoing the unswift transformation predicted by the Human Genome Project and the elusive breakthrough of cold fusion, must navigate a journey fraught with skepticism and lengthy timelines.
While some investors may be retreating as evidenced by Nvidia’s recent 30% stock decline from its peak, others see a silver lining. AI’s adoption is booming—71% of companies are now venturing into generative AI. Yet, despite such widespread experimentation, 80% of these organizations report no tangible earnings uplift. What’s stalling these expected gains? It’s not the AI technology; it’s us, the people expected to wield it.
Behavioural economics offers an explanation through the ambiguity effect, where decisions are stalled in the face of incomplete information. Companies find themselves tangled in trust issues, battling organizational inertia, and struggling with the cumbersome task of integrating AI into legacy systems. The flashing headlines may dazzle, but the profound shifts AI promises demand both patience and perseverance.
The experience of AI-human collaboration often reveals that unless carefully calibrated, these partnerships fall short. AI’s flair for pattern recognition must harmonize with human creativity and nuance. Only then can the promised productivity flourish. Yet, this ambitious synchronization hinges on trust—an all-too-fragile entity. Studies illuminate our quickness to abandon algorithms after perceived errors, even when they’re consistently right. Transparency and granting users some control can go a long way in maintaining faith in the system.
Businesses pushing forward will be those that prioritize building a trust-centric framework around their AI systems. These organizations will not only refine technology but will construct robust support systems where transparency and human collaboration dissolve the mystery of algorithms made inside black boxes.
Investors should, therefore, take a long view, prioritizing firms that focus on explanatory AI and human-AI cooperation over those promoting technological dazzle with little substance. Just as Warren Buffett advised investing with a clear-eyed view—shunning both blind optimism and outright skepticism—the wise path balances innovation with integration, ensuring that AI’s immense potential is carefully unleashed within systems poised to welcome it.
Ultimately, the true revolution begins not with AI’s capabilities, but with the trust we build and the pathways we create to harness it effectively. The future belongs to those who not only innovate but also instill confidence in the technology set to redefine industries.
The Hidden Truths About AI: What You Need to Know Before Diving In
Understanding the AI Terrain
Artificial intelligence (AI) is more than just a technology buzzword; it’s a transformative force capable of reshaping entire industries. From healthcare to finance, AI holds the promise of enhancing efficiency and driving innovation. However, as with any powerful tool, its integration into critical sectors must be handled with care to prevent unintended consequences. Here, we delve deeper into the current state of AI and unravel some of its less-explored facets.
Real-World Applications and Challenges
AI’s role in sectors like healthcare and finance is growing, yet hesitation lingers due to potential missteps that could have serious repercussions. To avoid catastrophic errors, these organizations must prioritize trust and reliability over rapid deployment.
How to Implement AI Effectively:
1. Understand Your Needs: Conduct a thorough needs analysis to determine how AI can best serve your organization.
2. Invest in Training: Educate your team about AI’s capabilities and limitations, fostering a culture of continuous learning.
3. Build a Trust-Centric Ecosystem: Ensure transparency in AI decisions and provide stakeholders with clear insights into AI processes.
4. Enhance Human-AI Collaboration: Pair AI’s pattern recognition with human creativity for superior outcomes.
5. Focus on Data Integrity: Reliable AI depends on high-quality, unbiased data. Regularly audit data sources for accuracy.
Market Trends and Predictions
Despite the struggles with adoption and trust, the AI market is projected to grow significantly. According to a report by Grand View Research, the AI market size was valued at USD 93.5 billion in 2021, and it is expected to expand at a compound annual growth rate (CAGR) of 38.1% from 2022 to 2030. Sectors like autonomous vehicles, smart manufacturing, and personalized medicine are flagged as key growth areas.
Controversies and Limitations
AI is not without its controversies. Concerns about job displacement, privacy issues, and ethical considerations are prevalent. The “black box” nature of many AI systems—where decision-making processes are not transparent—also raises alarms. Solving these issues requires:
– Development of Explainable AI Models: This involves creating algorithms whose decisions can be understood by humans.
– Strict Ethical Guidelines: Ethical AI development ensures fair, unbiased technology that safeguards user privacy and autonomy.
AI Features, Specs, and Pricing
The cost of implementing AI varies significantly based on the complexity and scale of the solution. Software as a Service (SaaS) AI platforms offer more affordable entry points, with costs primarily tied to usage levels and feature sets. Custom AI solutions, however, can cost well into the millions, including hardware, development, and maintenance expenses.
Security and Sustainability
AI’s environmental impact is a growing concern. The energy required for training large AI models is substantial, emphasizing the need for more sustainable approaches:
– Green AI Initiatives: Focus on energy-efficient algorithms and leveraging renewable energy resources.
– Responsible Data Management: Limit data storage to necessary information, reducing energy consumed by data centers.
Pros and Cons Overview
Pros:
– Enhanced efficiency and productivity
– Ability to process and analyze vast amounts of data
– Potential to drive innovation and new business models
Cons:
– Potential for bias and inaccuracy
– High initial costs for custom solutions
– Risk of over-reliance and job displacement
Conclusion and Quick Tips
To capitalize on AI’s potential, organizations should focus on building robust frameworks that prioritize trust, data integrity, and human-AI collaboration. Companies that strike the right balance between innovation and reliability will lead the charge in AI adoption.
Quick Tips:
– Prioritize AI tool transparency and user control to build trust.
– Regularly update AI models with fresh data to maintain accuracy.
– Encourage cross-disciplinary teams to maximize human and AI synergies.
For those interested in diving deeper into the AI landscape, exploring the offerings of AI leaders like Nvidia and IBM can provide valuable insights into cutting-edge developments.
Further Reading
Explore more about AI technologies and market trends at TechCrunch and Wired.