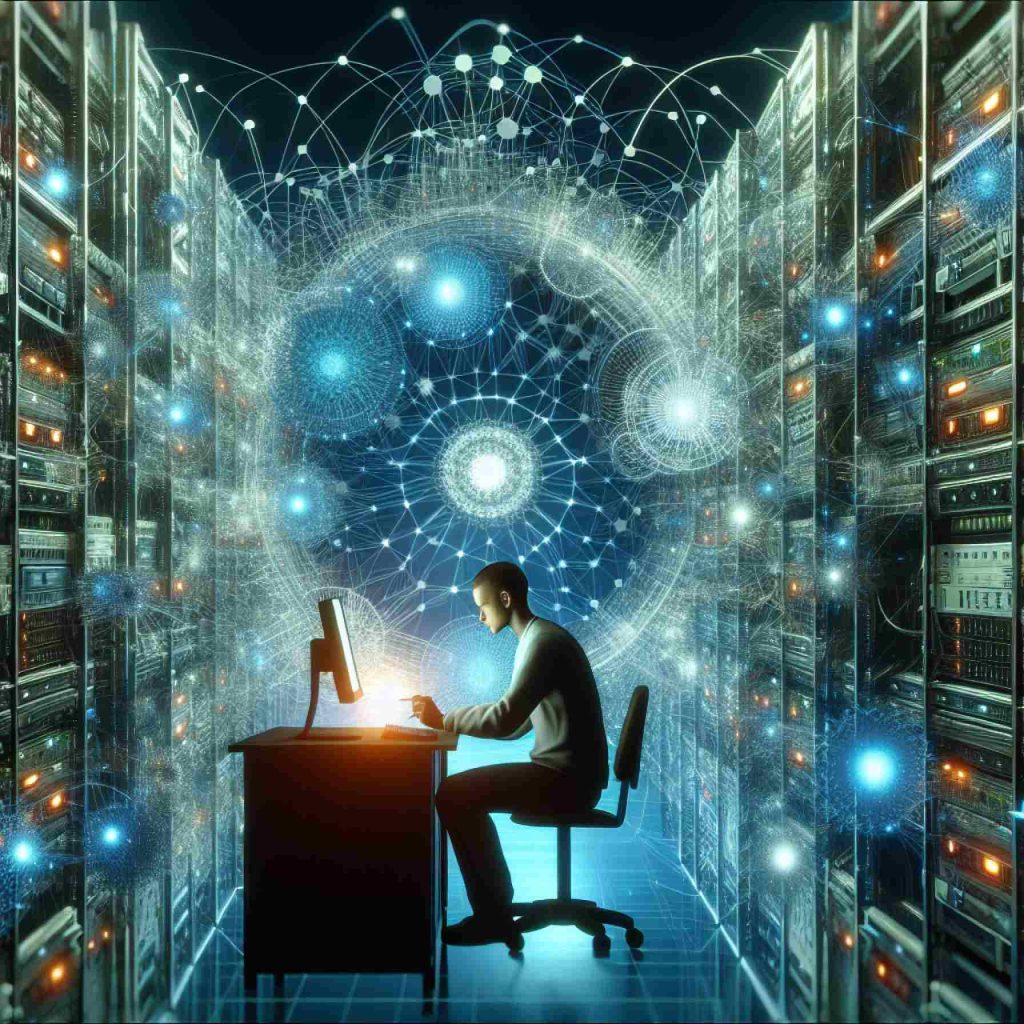
- Nihar Malali is transforming AI by integrating data privacy with technological progress.
- He received the “Best Paper Presenter” award at a leading IEEE conference for his work in privacy-focused AI.
- Malali utilizes federated learning, enabling AI training across decentralized devices while safeguarding data.
- His research with a Hybrid Convolutional Neural Network shows superior performance on the MNIST dataset, achieving 93.78% accuracy.
- His innovations align with increasing regulatory demands and consumer concerns over data privacy.
- Malali’s real-world experience as Principal Solutions Architect bridges academic and practical applications of AI.
- He advocates for “Glass-Box AI,” promoting transparency and ethical AI development.
- Malali’s work suggests a future where AI is compliant, human-centered, and enhances human creativity.
Nihar Malali is revolutionizing the landscape of artificial intelligence, fusing the intricate dance between data privacy and technological advancement. His latest triumph, a prestigious award for “Best Paper Presenter” at the 4th IEEE International Conference on Distributed Computing and Electrical Circuits and Electronics, acknowledges his groundbreaking work in creating AI that respects user privacy.
In the digital tapestry of our times, where data breaches loom like shadows over innovation, Malali has threaded a silver lining. He harnesses the power of federated learning, a method where machine learning models are trained across a myriad of decentralized devices, protecting the sanctity of the data they contain. Imagine it as conducting an orchestra without ever gathering the individual musicians in a single hall – the symphony is created, yet each instrument remains sovereign.
Diving deep into the world of image classification, Malali’s research pitted a Hybrid Convolutional Neural Network against traditional models, revealing a prowess unmatched and untethered to increased risks. On the revered MNIST dataset, his model achieved an impressive 93.78% accuracy, soaring above its counterparts like the Multilayer Perceptron and the Vision Transformer. It’s not just numbers; it’s a story of proving that privacy and performance can indeed waltz together.
The timing of this innovation couldn’t be more apt. With regulatory scrutiny tightening its grip and consumers increasingly protective of their digital footprints, Malali’s work offers a beacon of hope. Industries ranging from healthcare to finance can now envision a future where collaborative, privacy-conscious AI helps them soar beyond the boundaries of traditional data-sharing frameworks. Through this, organizations can craft more resilient, compliant, and ultimately human-centered AI solutions.
Beyond the paper, Malali’s journey is a testament to bridging the ever-so-often gap between academic rigor and real-world application. As Principal Solutions Architect at National Life Group, his real-world experience infuses his research with pragmatism and translatability, setting the stage for future innovations that marry ethical AI with robust performance.
But this narrative is more than a tale of achievement; it’s a paradigm shift towards “Glass-Box AI,” where transparency and interpretability are not just idealistic aspirations but essential principles. Malali envisions a world where AI enhances rather than replaces human ingenuity—a world where technology’s light guides rather than blinds.
This accolade not only places Malali among the elite circles of AI thought leaders but more profoundly emphasizes the vital dialogue between privacy and progress. As we tread into the future, one thing is clear—Nihar Malali is not just shaping AI; he’s ensuring its heart beats in time with the values we cherish.
This AI Revolution is Changing Everything: Meet the Innovator Behind Privacy-Focused Machine Learning
The Intersection of AI Innovation and Data Privacy
Nihar Malali is at the forefront of a transformative movement in artificial intelligence (AI), addressing the often conflicting goals of data privacy and technological advancement. His key achievement—the introduction of a novel approach to federated learning—has earned him the prestigious “Best Paper Presenter” award at the 4th IEEE International Conference on Distributed Computing and Electrical Circuits and Electronics. Let’s delve deeper into this innovation and explore its potential impacts across various sectors.
How Federated Learning Enhances AI
Federated learning represents a breakthrough in AI, allowing models to be trained on decentralized devices without moving sensitive data to a central server. This approach not only improves privacy but also reduces the risks associated with data breaches. By maintaining data on the device where it originates, federated learning adheres to modern privacy regulations like GDPR in Europe and CCPA in California.
How-To Steps for Implementing Federated Learning:
1. Device Preparation: Ensure all devices involved in the learning process have necessary computational resources.
2. Model Initialization: Distribute a global model to each device to start the learning process.
3. Local Training: Train the model on each device using local data.
4. Model Aggregation: Collect the locally trained models and aggregate them to update the global model without sharing raw data.
5. Model Deployment: Deploy the updated model back to the devices for further learning and refinement.
Real-World Use Cases
Malali’s research, particularly his work with a Hybrid Convolutional Neural Network, demonstrates exceptional performance on image classification tasks. On the MNIST dataset, his model achieved a 93.78% accuracy, showcasing the potential of privacy-preserving AI in fields such as:
– Healthcare: Hospitals can leverage federated learning to improve diagnostic algorithms without breaching patient confidentiality.
– Finance: Banks can enhance fraud detection systems by learning from transaction data in a decentralized manner.
– Smart Cities: Federated learning can optimize traffic management systems by using data from distributed sensors.
Challenges and Limitations
Despite its promising benefits, federated learning presents challenges, such as:
– Communication Overhead: Frequent updates between devices can strain network bandwidth.
– Heterogeneity: Differing computational power and data quality across devices can affect performance consistency.
Expert Insights and Predictions
Nihar Malali’s approach aligns with the current industry trend of shifting towards transparent and ethical AI. As regulatory pressures mount, companies will need to embrace these principles, making federated learning an increasingly critical component of AI strategies.
– Security and Sustainability: By decentralizing data processing, federated learning minimizes attack vectors, making data more secure. It also conserves energy by processing data closer to its source.
Actionable Tips
Organizations looking to integrate privacy-conscious AI should:
– Start Small: Pilot federated learning projects on non-critical data to evaluate applicability.
– Invest in Infrastructure: Ensure devices can handle the computational load of local model training.
– Stay Informed: Keep up with regulatory changes to ensure compliance.
Conclusion
Nihar Malali’s innovative approach is more than an academic exercise; it represents a crucial step towards reconciling privacy with AI performance. As industries worldwide grapple with data privacy concerns, his work serves as a beacon, guiding them towards sustainable and secure AI solutions.
For further information on federated learning and its implications, explore more at the [IEEE](https://www.ieee.org) and [AI Ethics](https://www.aai.com).
Suggested Related Domains
– [IEEE](https://www.ieee.org) for conferences and publications on cutting-edge technology
– [AI Ethics](https://www.aai.com) for insights into ethical considerations in AI development