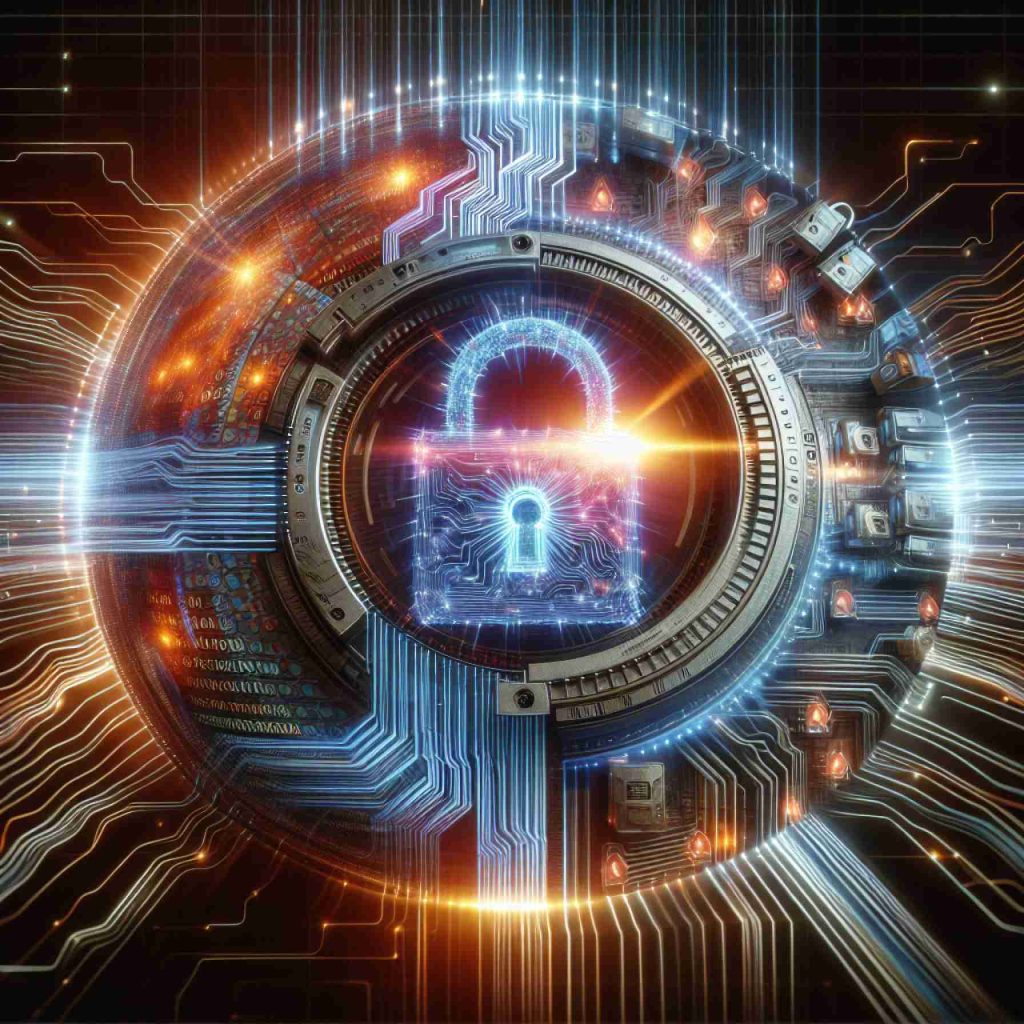
- Nihar Malali, Principal Solutions Architect at National Life Group, is recognized for integrating AI innovation with data privacy.
- His research at the 4th IEEE International Conference focuses on leveraging AI through federated learning to enhance privacy.
- Federated learning allows AI to train models across decentralized devices, reducing the risk of data breaches.
- Malali’s Hybrid Convolutional Neural Networks achieve 93.78% accuracy on the MNIST dataset, outperforming traditional models while preserving privacy.
- This approach is crucial as regulatory scrutiny increases in industries like finance and healthcare.
- Malali advocates for “glass-box AI,” emphasizing transparency and human-machine collaboration.
- His work supports responsible AI development, aligning with ethical standards and enhancing trust.
- Malali’s framework offers a path for industries to advance AI technologies without compromising user data privacy.
As the digital landscape transforms and the demand for robust data privacy intensifies, one name has surged to prominence on the intersection of artificial intelligence and privacy concerns: Nihar Malali. The inventive Principal Solutions Architect at National Life Group has recently carved a niche in the tech world by melding complex AI technology with pressing privacy needs. Celebrated at the 4th IEEE International Conference on Distributed Computing and Electrical Circuits and Electronics, Malali’s award-winning research challenges conventional paradigms, placing privacy at the forefront of AI innovation.
Malali’s research, articulated through his pioneering paper, deciphers a contemporary conundrum—how to leverage AI’s transformative power while safeguarding user data. He explores this issue through the lens of federated learning, a cutting-edge approach that empowers AI algorithms to train across decentralized devices. This minimizes the dangers of privacy infringements, a response to international anxiety over data breaches and regulatory oversight.
Visualize the Hybrid Convolutional Neural Networks (CNNs) developed by Malali as a fortress against unwanted data exposure. His model, meticulously tested on the MNIST dataset, attained an impressive accuracy rate of 93.78%. This triumph over traditional architectures like the Multilayer Perceptron and Vision Transformer repositions the possibilities of image classification, ensuring superior performance while preserving privacy.
The stakes are higher as regulatory scrutiny sharpens across industries from finance to healthcare. In this climate, federated learning’s rise is timely. Malali offers a blueprint, hewn from theoretical rigor and practical application, that enables industries to cultivate superior predictive models without compromising on user data.
Picture healthcare institutions, arm-in-arm, crafting enhanced diagnostic tools without sacrificing patient privacy. Or financial giants refining their fraud detection systems in compliance with stringent guidelines. Malali’s framework paves the way for secure collaboration, aligning technological advancement with society’s ethical demands.
Underpinning his work is an unwavering commitment to “glass-box AI”—transparent systems that champion collaboration between humans and machines, rather than supplanting human roles. Such systems promise accountability and compliance, crucial as AI’s influence permeates every facet of life.
This accolade from the IEEE conference isn’t merely a trophy for Malali; it’s a testament to the broader implications his work holds for responsible AI evolution. Those at the vanguard of technology adoption, from industry leaders to policymakers, will find his methodologies critical in navigating the treacherous waters of data privacy.
Malali’s innovation is not just a footnote in the annals of AI development. It is a clarion call for a future where AI respects human dignity, aligns with ethical standards, and empowers us all towards a more secure digital tomorrow. With trailblazers like Malali leading the charge, the intersection of AI and privacy promises not just technological revolution, but an evolution of trust and security.
How Federated Learning and AI Privacy Are Shaping the Future
Understanding Malali’s Groundbreaking Work
The intersection of artificial intelligence (AI) and data privacy is increasingly pressing as technology pervades every aspect of life. Nihar Malali’s pioneering efforts in this domain focus on addressing privacy concerns using federated learning, a decentralized approach ensuring that sensitive user data remains confidential and secure.
What is Federated Learning?
Federated learning is a machine learning technique that enables algorithms to be trained across multiple decentralized devices or servers holding local data samples. This innovative approach allows for the development of AI models without transferring user data to central servers, significantly reducing the risk of data breaches and privacy violations.
Federated learning’s methodology protects user data by training directly on the device’s data and only sending model updates rather than raw data to a central server. This process is crucial in environments with stringent data privacy concerns, such as finance and healthcare.
The Role of Hybrid CNNs in Data Privacy
Malali’s work with Hybrid Convolutional Neural Networks (CNNs) exemplifies the cutting-edge of this technology, achieving an impressive accuracy rate of 93.78% on benchmark tests. This model surpasses traditional neural network architectures, demonstrating higher performance levels while maintaining robust privacy protection.
Real-World Applications
Healthcare: Federated learning can help hospitals collaborate to improve diagnostic tools using collective data insights, without overstepping privacy boundaries related to patient data.
Finance: The financial sector could leverage these techniques to enhance fraud detection systems, ensuring compliance with regulatory measures while protecting client information.
Industry Trends and Predictions
With privacy regulations tightening globally, federated learning is poised to grow significantly. Gartner predicts that by 2025, 60% of organizations that use machine learning will leverage federated or distributed ML workflows. The rising demand for privacy-preserving AI solutions will spur investment and innovation in federated learning.
Pros and Cons Overview
Pros:
– Enhanced Privacy: User data stays on their devices, minimizing data exposure risks.
– Compliance: Federated learning aligns well with data protection regulations.
– Scalability: Applicable across sectors, from healthcare to retail, without large-scale data transfers.
Cons:
– Complexity: Implementing federated learning systems can be more complex than traditional AI models.
– Communication Overhead: Requires robust communication infrastructure between devices.
– Limited Data Utilization: May not access the full benefits of centralized, large-scale data analysis.
Actionable Recommendations
– Adopt Federated Learning: Organizations should consider implementing federated learning to enhance privacy and data security.
– Invest in Infrastructure: Building a solid infrastructure to support decentralized learning is crucial.
– Stay Informed: Keep abreast of emerging regulations and AI technology trends to maintain compliance and leverage new opportunities.
Conclusion
Nihar Malali’s work exemplifies the potential of integrating AI with strong privacy frameworks, setting a new industry standard. As organizations navigate the complexities of AI adoption, understanding and implementing federated learning can ensure compliance and foster trust with clients and users.
For further insights into AI and federated learning innovations, explore tech giant Google’s initiatives in this emerging field.