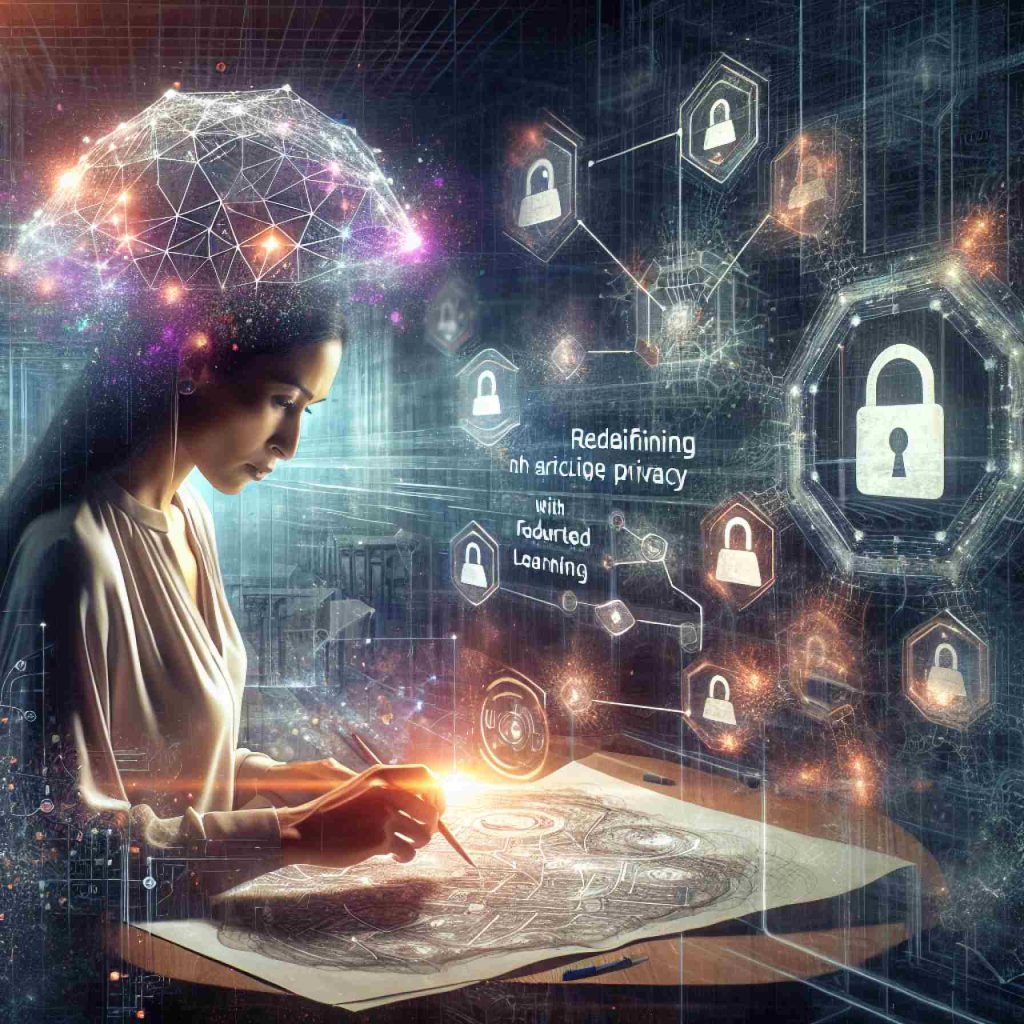
- Nihar Malali, Principal Solutions Architect at National Life Group, integrates data privacy with AI innovation, exemplifying future AI that emphasizes user privacy.
- His work with federated learning—a decentralized approach allowing algorithm refinement without data exchange—highlights advancements in data security.
- Malali’s Hybrid Convolutional Neural Networks achieve 93.78% accuracy on the MNIST dataset, surpassing traditional models like Multilayer Perceptrons and Vision Transformers.
- His research is timely, offering privacy-conscious AI solutions vital for industries such as healthcare and finance under regulatory scrutiny.
- At National Life Group, Malali applies AI in predictive mortality modeling and fraud detection, merging theoretical insights with practical applications.
- He advocates for “Glass-Box AI,” emphasizing transparency, accountability, and ethical alignment with human values, supporting AI as a complement to human intelligence.
- His recognition at the IEEE conference embodies his role in guiding the ethical, innovative integration of AI into society.
Bold strokes of innovation define Nihar Malali’s journey as a Principal Solutions Architect at the National Life Group, where he skillfully merges data privacy with AI’s expansive promise. Poised at the intersection of technological advancement and ethical responsibility, Malali’s work exemplifies the future of artificial intelligence that fiercely protects user privacy.
His recent recognition as the “Best Paper Presenter” at the IEEE International Conference on Distributed Computing showcases his pioneering spirit. His profound insights into federated learning, detailed in a compelling paper, capture the imagination. Federated learning, a decentralized method where algorithms refine their accuracy across numerous servers without the traditional requirement of data exchange, becomes the hero in the ongoing saga of data security.
Malali’s work specifically hones in on Hybrid Convolutional Neural Networks (CNNs) to push the envelope in image classification. With an impressive 93.78% accuracy achieved on the MNIST dataset, his Hybrid CNN outshines traditional competitors like Multilayer Perceptrons and Vision Transformers, revealing a pathway to more secure AI applications.
The timing of this research could not be more critical. As privacy-regulating scrutiny mounts, stakeholders in industries from healthcare to finance seek robust yet respectful AI solutions. Enterprises keen on safeguarding sensitive data without forsaking analytical prowess find a blueprinted solution in Malali’s research.
Nihar Malali does not merely rest on theoretical laurels. Innovative strategies permeate his efforts at National Life Group, where he integrates AI into everything from predictive mortality modeling to dynamic fraud detection systems. This blend of academia and practical application illustrates a roadmap for enterprises aiming to adopt AI without compromising ethics or compliance.
The broader implications? Significant, to say the least. Imagine a world where hospitals share knowledge to diagnose diseases better, yet patient confidentiality remains unbreachable; or where banks could ramp up fraud prevention, all while adhering to rigorous data protection laws. Federated learning, as envisioned by Malali, makes these scenarios vividly plausible.
Looking beyond, his elements are a testament to the drive for “Glass-Box AI”—a transparent, comprehensible framework that ensures AI remains accountable and aligned with human values. Malali champions AI as a complementary force to human intelligence, not a replacement, reinforcing the need for collaboration in a digitally-driven future.
The accolade from IEEE is more than a personal milestone. It marks Malali’s place among global technology visionaries dedicated to bridging the divide between scientific exploration and industry application. As AI navigates towards increasingly complex challenges, his work is a beacon guiding the safe, innovative, and ethical integration of AI into our world.
Nihar Malali’s dedication to preserving the integrity of AI while propelling it forward marks a significant stride towards a balanced symbiosis of technology and human values. His evolving narrative invites us all to ponder the possibilities of a world where AI respects our digital boundaries while enhancing our collective potential.
Revolutionizing AI: How Nihar Malali Merges Data Privacy with Cutting-Edge Technology
Nihar Malali stands at the forefront of a digital revolution, exemplifying a rare blend of technical acumen and ethical commitment. As a Principal Solutions Architect at National Life Group, his groundbreaking work navigates the challenging waters of integrating data privacy with AI innovation. Let’s delve deeper into the impact of his work and explore facets that can guide enterprises keen on tapping into AI’s potential while upholding privacy.
More on Federated Learning and Hybrid CNNs
1. Federated Learning: A Paradigm Shift
– Federated learning fundamentally alters how AI models are trained by allowing data to remain on local servers while only model updates are shared globally. This approach significantly reduces the risk of exposing sensitive data during the training process. [Forbes](https://forbes.com) discusses how this model caters exceedingly well to privacy-conscious industries like healthcare and finance.
2. Hybrid Convolutional Neural Networks (CNNs) Advantages
– By achieving a remarkable 93.78% accuracy on the MNIST dataset, Malali’s Hybrid CNN showcases superior performance over traditional models. Hybrid CNNs leverage convolutional layers to capture spatial hierarchies in data, providing enhanced image classification capabilities. Their application is poised to transform fields like autonomous driving and medical imaging by offering efficient and accurate predictions with lesser data.
Pressing Industry Questions Answered
– How does Malali’s work influence data privacy regulations?
– With increasing scrutiny from regulations like GDPR and CCPA, federated learning presents a compliant method for AI training. It aids organizations in maintaining privacy without sacrificing data analytics’ competitive edge.
– What are the real-world applications of Malali’s AI strategies?
– In sectors like healthcare, banks, and insurance, federated learning can enable secure data collaboration. Hospitals can collaborate to identify health trends without exposing patient data, while banks can enhance fraud detection by securely analyzing transaction data. [National Life Group](https://nationallife.com) actively seeks ways to incorporate such innovations effectively.
Industry Trends and Predictions
– Growth in Federated Learning
– Research suggests that the federated learning market is set to grow exponentially, potentially reaching a valuation of several billion by 2028. This growth is driven by increasing investment in privacy-preserving AI solutions. McKinsey predicts this trend will significantly impact data-dependent industries, offering secure yet powerful AI tools.
Pros and Cons Overview
– Pros
– Enhances privacy protection
– Complies with data regulations
– Facilitates collaborative learning across diverse datasets
– Cons
– High communication costs
– Complexity in managing distributed datasets
– Early-stage in industry-standard adoption
Quick Tips for Implementation
1. Analyze Data Requirements: Assess if federated learning aligns with your business’s data privacy and analytical needs before integration.
2. Pilot Small Projects: Begin with pilot implementations in controlled environments to evaluate performance and compliance with privacy regulations.
3. Stay Updated with Regulatory Changes: Continuously monitor legal requirements around data privacy to ensure ongoing compliance.
Conclusion
Nihar Malali’s pioneering work elucidates a future where AI respects personal data boundaries while driving innovation. The embrace of federated learning marks a decisive step towards achieving this balance. Enterprises eager to leverage AI must consider adopting similar strategies to remain competitive and compliant in an increasingly regulated landscape.
For additional insights into Malali’s work and federated learning, visit National Life Group and Forbes.