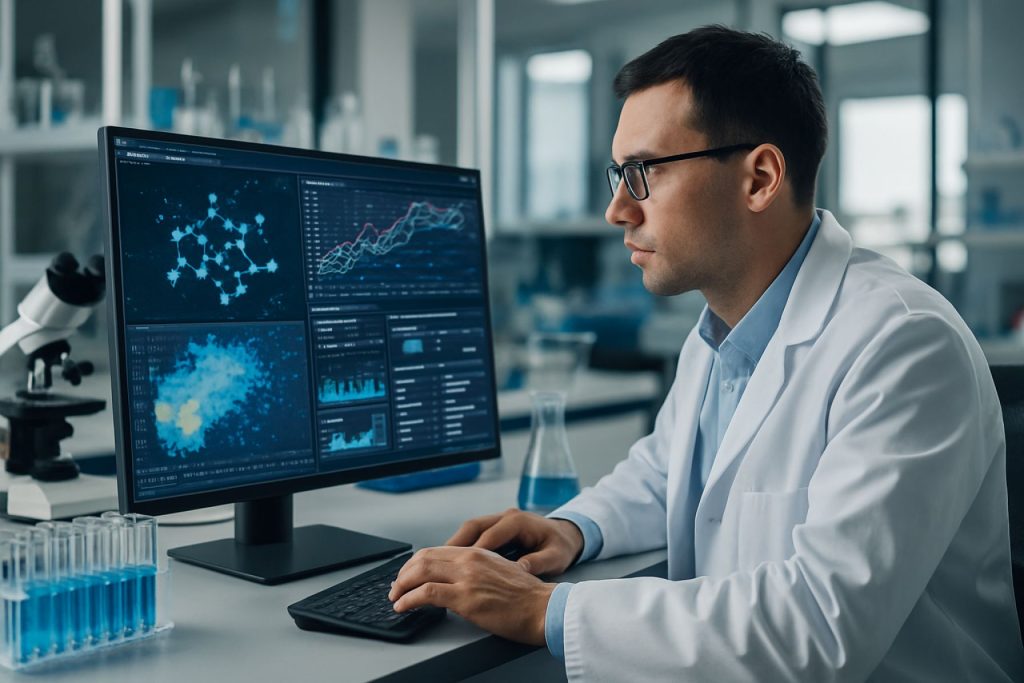
How AI-Driven Scientific Data Analysis Platforms Are Revolutionizing Research in 2025: Market Acceleration, Key Technologies, and the Road Ahead. Discover the Transformative Impact of AI on Scientific Discovery and Data Insights.
- Executive Summary: Key Findings and Market Highlights
- Market Size and Growth Forecast (2025–2030): CAGR and Revenue Projections
- Core Technologies Powering AI-Driven Scientific Data Analysis
- Competitive Landscape: Leading Platforms and Innovators
- Adoption Trends Across Scientific Disciplines
- Integration with Laboratory and Research Workflows
- Data Security, Compliance, and Ethical Considerations
- Case Studies: Real-World Impact and Breakthroughs
- Challenges, Barriers, and Opportunities for Stakeholders
- Future Outlook: Emerging Trends and Strategic Recommendations
- Sources & References
Executive Summary: Key Findings and Market Highlights
AI-driven scientific data analysis platforms are rapidly transforming research and innovation across multiple scientific domains in 2025. These platforms leverage advanced machine learning, deep learning, and natural language processing to automate data processing, accelerate discovery, and enhance reproducibility. The integration of AI into scientific workflows is enabling researchers to handle increasingly complex and voluminous datasets, driving breakthroughs in fields such as life sciences, materials science, and environmental research.
Key industry leaders, including IBM, Microsoft, and Google, are expanding their AI-powered cloud platforms to support scientific research. IBM’s Watson platform, for example, is being utilized in genomics and drug discovery, offering automated data curation and hypothesis generation. Microsoft’s Azure AI services are increasingly adopted by research institutions for scalable data analysis and collaboration. Google Cloud’s AI and machine learning tools are supporting large-scale scientific projects, including climate modeling and protein structure prediction.
Specialized providers are also making significant contributions. Elsevier and Thermo Fisher Scientific are integrating AI into their scientific data management and laboratory informatics solutions, enabling automated data annotation, pattern recognition, and predictive analytics. PerkinElmer is advancing AI-driven platforms for laboratory automation and multi-omics data analysis, while Sartorius is focusing on AI-powered bioprocess data analytics.
In 2025, the adoption of AI-driven platforms is being propelled by the need for faster, more accurate insights from complex datasets, as well as the growing emphasis on open science and data sharing. The convergence of AI with cloud computing and high-performance computing infrastructure is lowering barriers to entry for research organizations of all sizes. Regulatory agencies and scientific bodies are increasingly recognizing the value of AI in ensuring data integrity and reproducibility.
Looking ahead, the next few years are expected to see further integration of generative AI, explainable AI, and automated experiment design into scientific data analysis platforms. Collaboration between technology providers, research institutions, and industry consortia will be critical in addressing challenges related to data privacy, standardization, and ethical AI use. The market outlook remains robust, with AI-driven platforms poised to become foundational tools for scientific discovery and innovation worldwide.
Market Size and Growth Forecast (2025–2030): CAGR and Revenue Projections
The market for AI-driven scientific data analysis platforms is poised for robust expansion between 2025 and 2030, propelled by the accelerating adoption of artificial intelligence across research-intensive sectors such as pharmaceuticals, materials science, genomics, and environmental studies. As of 2025, the global market is estimated to be valued in the low-to-mid single-digit billions (USD), with leading industry participants reporting significant year-over-year growth in both platform adoption and recurring software revenues.
Key players in this space include IBM, whose Watson platform is widely used for AI-powered data analysis in life sciences and healthcare research, and Thermo Fisher Scientific, which integrates AI into its cloud-based informatics solutions for laboratory data management and analysis. Siemens is also notable for its AI-driven platforms supporting scientific and industrial R&D, while PerkinElmer and Agilent Technologies are expanding their AI-enabled informatics offerings for chemical and biological data analysis.
The compound annual growth rate (CAGR) for the sector is projected to range between 18% and 25% through 2030, reflecting both the increasing volume and complexity of scientific data and the growing demand for automation and advanced analytics in research workflows. This growth is underpinned by the rapid evolution of AI algorithms, improved cloud infrastructure, and the proliferation of high-throughput experimental technologies that generate vast datasets requiring sophisticated analysis.
By 2030, the market is expected to surpass the USD 10 billion mark, with some forecasts suggesting even higher valuations as AI becomes further embedded in scientific discovery pipelines. The pharmaceutical and biotechnology industries are anticipated to remain the largest end-users, leveraging AI-driven platforms for drug discovery, clinical trial optimization, and biomarker identification. However, significant uptake is also expected in materials science, environmental monitoring, and academic research, as organizations seek to accelerate innovation cycles and extract actionable insights from complex, multi-modal datasets.
Strategic investments and partnerships are likely to intensify over the next few years, with established technology providers such as Microsoft and Google (via their cloud and AI divisions) entering or expanding their presence in the scientific data analysis domain. This competitive landscape is expected to drive further innovation, lower barriers to adoption, and broaden the accessibility of AI-driven scientific data analysis platforms worldwide.
Core Technologies Powering AI-Driven Scientific Data Analysis
AI-driven scientific data analysis platforms are rapidly transforming the landscape of research and innovation by leveraging advanced computational techniques to extract insights from complex datasets. As of 2025, these platforms are underpinned by a convergence of core technologies, including machine learning (ML), deep learning, natural language processing (NLP), and scalable cloud infrastructure. The integration of these technologies is enabling researchers to accelerate discovery, automate data interpretation, and foster interdisciplinary collaboration.
A central pillar of these platforms is the use of sophisticated ML and deep learning algorithms, which can identify patterns and relationships in high-dimensional scientific data. For example, IBM’s Watson platform employs AI models to analyze genomics, materials science, and chemical data, supporting hypothesis generation and experimental design. Similarly, Microsoft’s Azure AI services provide scalable tools for processing and interpreting large-scale scientific datasets, with applications ranging from climate modeling to drug discovery.
Natural language processing is another critical technology, enabling platforms to parse and synthesize vast volumes of scientific literature and unstructured data. Google’s AI research division has developed models capable of extracting relevant information from millions of research articles, facilitating literature reviews and knowledge graph construction. These capabilities are increasingly integrated into platforms to help scientists stay abreast of the latest findings and contextualize their own results.
Cloud computing infrastructure is essential for the scalability and accessibility of AI-driven analysis. Providers such as Amazon (through AWS) and Oracle offer robust environments for running intensive AI workloads, supporting collaborative research across institutions and geographies. These infrastructures enable the deployment of containerized AI models, real-time data streaming, and secure data sharing, all of which are vital for modern scientific workflows.
Looking ahead, the next few years are expected to see further integration of quantum computing and edge AI into scientific data analysis platforms. Companies like IBM and Intel are actively developing quantum and neuromorphic computing technologies that promise to tackle currently intractable scientific problems. Additionally, the adoption of open-source frameworks and standardized data formats is anticipated to enhance interoperability and reproducibility, addressing key challenges in scientific research.
In summary, the core technologies powering AI-driven scientific data analysis platforms in 2025 are enabling unprecedented advances in research productivity and insight generation. As these technologies mature and converge, they are set to redefine the boundaries of scientific discovery in the coming years.
Competitive Landscape: Leading Platforms and Innovators
The competitive landscape for AI-driven scientific data analysis platforms in 2025 is marked by rapid innovation, strategic partnerships, and increasing adoption across academia, pharmaceuticals, materials science, and life sciences. Several established technology giants and specialized startups are vying for leadership, leveraging advances in machine learning, natural language processing, and cloud computing to transform how scientific data is processed and interpreted.
Among the most prominent players, IBM continues to expand its AI-powered data analysis offerings through its Watson platform, which is widely used in genomics, drug discovery, and materials research. IBM’s focus on explainable AI and integration with high-performance computing resources has positioned it as a preferred partner for large-scale scientific collaborations. Similarly, Microsoft has made significant strides with its Azure AI platform, providing scalable tools for data ingestion, analysis, and visualization tailored to scientific research. Microsoft’s partnerships with research institutions and pharmaceutical companies have accelerated the deployment of AI models for tasks such as protein structure prediction and clinical trial optimization.
In the life sciences sector, Thermo Fisher Scientific has integrated AI-driven analytics into its cloud-based laboratory informatics solutions, enabling researchers to automate data processing from instruments and derive actionable insights more efficiently. PerkinElmer is another key player, offering platforms that combine AI with multi-omics data analysis, supporting breakthroughs in personalized medicine and biomarker discovery.
Startups and scale-ups are also shaping the competitive landscape. DeepMind, a subsidiary of Alphabet, has made headlines with its AlphaFold system, which uses AI to predict protein structures with unprecedented accuracy, revolutionizing structural biology. Meanwhile, Benchling provides cloud-based R&D platforms enhanced with AI capabilities for data management and analysis, serving a growing base of biotech and pharmaceutical clients.
Looking ahead, the next few years are expected to see intensified competition as companies race to integrate generative AI and foundation models into scientific workflows. The convergence of AI with quantum computing, as explored by IBM and Microsoft, could further accelerate discovery cycles. Additionally, open-source initiatives and collaborations between industry and academia are likely to democratize access to advanced AI tools, fostering innovation and expanding the market for AI-driven scientific data analysis platforms.
Adoption Trends Across Scientific Disciplines
The adoption of AI-driven scientific data analysis platforms is accelerating across a broad spectrum of scientific disciplines in 2025, driven by the exponential growth of research data and the need for advanced analytics. In life sciences, platforms leveraging machine learning and deep learning are now integral to genomics, drug discovery, and clinical research. For example, Illumina has integrated AI into its sequencing workflows, enabling faster and more accurate genomic data interpretation. Similarly, Thermo Fisher Scientific offers AI-powered solutions for proteomics and high-throughput screening, streamlining the identification of biomarkers and therapeutic targets.
In chemistry and materials science, AI platforms are transforming the way researchers analyze complex datasets from spectroscopy, crystallography, and molecular simulations. IBM has expanded its AI-driven platform capabilities, such as IBM Watson, to support materials discovery and chemical synthesis prediction, facilitating rapid innovation in battery technology and sustainable materials. The integration of AI with laboratory automation is also gaining traction, with companies like PerkinElmer deploying AI-enhanced informatics platforms to manage and interpret large-scale experimental data.
Environmental sciences are witnessing increased adoption of AI platforms for climate modeling, remote sensing, and ecological monitoring. Esri, a leader in geographic information systems, has embedded AI and machine learning into its ArcGIS platform, enabling researchers to analyze satellite imagery and environmental data at unprecedented scales. These capabilities are crucial for tracking climate change impacts, biodiversity loss, and natural resource management.
Physics and astronomy are also benefiting from AI-driven data analysis, particularly in the management of data from large-scale experiments and observatories. CERN employs AI algorithms to process petabytes of data generated by the Large Hadron Collider, accelerating the identification of rare particle events. In astronomy, AI platforms are used to automate the classification of celestial objects and detect transient phenomena in real time.
Looking ahead, the outlook for AI-driven scientific data analysis platforms is robust. Interdisciplinary collaboration is expected to intensify, with platforms becoming more interoperable and customizable to specific research needs. The integration of generative AI and foundation models is anticipated to further enhance hypothesis generation and experimental design. As data volumes continue to surge, the adoption of these platforms is poised to become ubiquitous across scientific domains, fundamentally reshaping research methodologies and accelerating discovery.
Integration with Laboratory and Research Workflows
The integration of AI-driven scientific data analysis platforms with laboratory and research workflows is accelerating rapidly in 2025, driven by the need for higher throughput, reproducibility, and actionable insights from increasingly complex datasets. Modern laboratories are leveraging AI to automate data processing, enhance experimental design, and facilitate real-time decision-making, fundamentally transforming the research landscape.
A key trend is the seamless connection between laboratory instruments, data management systems, and AI analytics engines. Leading laboratory automation providers such as Thermo Fisher Scientific and Agilent Technologies are embedding AI modules directly into their informatics platforms. These integrations enable automated data capture from instruments, immediate preprocessing, and advanced analytics—such as anomaly detection, pattern recognition, and predictive modeling—without manual intervention. For example, Thermo Fisher’s cloud-based laboratory information management systems (LIMS) now offer AI-powered modules for sample tracking, quality control, and workflow optimization, reducing human error and accelerating research cycles.
In pharmaceutical and life sciences research, AI-driven platforms are being integrated with electronic lab notebooks (ELNs) and laboratory execution systems (LES) to streamline experiment documentation and compliance. PerkinElmer and Waters Corporation have expanded their digital laboratory ecosystems to include AI-based data analysis tools that automatically interpret results, suggest next steps, and flag inconsistencies. This integration is particularly valuable in multi-omics, drug discovery, and clinical research, where data volume and complexity can overwhelm traditional analysis methods.
Another significant development is the adoption of open, interoperable platforms that facilitate integration across diverse laboratory environments. Sartorius and Bruker are advancing modular AI solutions that can connect with third-party instruments and software, supporting collaborative research and data sharing. These platforms often leverage standardized data formats and APIs, enabling researchers to build custom workflows that incorporate AI-driven analytics at every stage—from sample preparation to result interpretation.
Looking ahead, the next few years are expected to see further convergence of AI, automation, and cloud technologies in laboratory settings. The proliferation of edge AI—where data is processed locally on laboratory devices—will enable real-time feedback and adaptive experimentation. As regulatory bodies increasingly recognize the value of AI in ensuring data integrity and reproducibility, integration with compliance and audit systems will become standard. Collectively, these advances are poised to make AI-driven data analysis an indispensable component of modern laboratory and research workflows.
Data Security, Compliance, and Ethical Considerations
As AI-driven scientific data analysis platforms become increasingly central to research and innovation, data security, compliance, and ethical considerations are at the forefront of industry and regulatory discussions in 2025. The integration of advanced machine learning and large language models into scientific workflows has amplified both the opportunities and risks associated with sensitive data handling, particularly in fields such as genomics, pharmaceuticals, and materials science.
Major platform providers, including IBM, Microsoft, and Google, have responded by embedding robust security frameworks into their AI research platforms. These frameworks typically include end-to-end encryption, granular access controls, and continuous monitoring for anomalous activity. For example, IBM’s Watsonx platform emphasizes secure data enclaves and federated learning, allowing sensitive datasets to remain on-premises while still benefiting from AI-driven insights.
Compliance with evolving regulations is a critical concern. The European Union’s AI Act, expected to be fully enforced by 2025, imposes strict requirements on transparency, data provenance, and risk management for AI systems used in scientific research. Leading platforms are adapting by providing detailed audit trails, explainable AI features, and automated compliance reporting. Microsoft’s Azure AI services, for instance, offer built-in compliance certifications and tools to help organizations meet GDPR, HIPAA, and other international standards.
Ethical considerations are also shaping platform development. There is growing emphasis on bias mitigation, reproducibility, and responsible data stewardship. Google’s AI research division has published open-source toolkits for fairness assessment and model interpretability, which are being integrated into cloud-based scientific analysis suites. Additionally, industry consortia such as the LF AI & Data Foundation are promoting open standards and best practices for ethical AI deployment in scientific contexts.
Looking ahead, the next few years will likely see increased collaboration between platform providers, research institutions, and regulatory bodies to establish interoperable security protocols and shared ethical guidelines. The convergence of AI, cloud computing, and secure data management is expected to accelerate scientific discovery while ensuring that privacy, compliance, and ethical imperatives remain central to platform evolution.
Case Studies: Real-World Impact and Breakthroughs
AI-driven scientific data analysis platforms are rapidly transforming research and development across multiple scientific domains. In 2025, several high-profile case studies highlight the tangible impact and breakthroughs enabled by these technologies, particularly in pharmaceuticals, materials science, and climate research.
One of the most prominent examples is the use of IBM‘s Watson platform in drug discovery. In collaboration with leading pharmaceutical companies, Watson’s AI models have accelerated the identification of novel drug candidates by analyzing vast datasets of molecular structures, clinical trial results, and scientific literature. In 2024 and 2025, Watson’s AI-driven insights contributed to the rapid development of new antiviral compounds, reducing the typical lead identification phase from years to months. This success is attributed to Watson’s ability to integrate heterogeneous data sources and generate actionable hypotheses, which are then validated in laboratory settings.
In materials science, Microsoft has advanced its Azure Quantum platform, integrating AI with quantum-inspired algorithms to analyze complex materials datasets. In 2025, researchers at major universities and industrial partners used Azure Quantum to discover new battery materials with higher energy densities and improved safety profiles. The platform’s AI models sifted through millions of potential chemical combinations, predicting properties and performance metrics with high accuracy. This approach has led to the identification of several promising candidates now entering pilot-scale production, demonstrating the platform’s value in accelerating innovation cycles.
Climate science has also benefited from AI-driven platforms. Google has deployed its AI-based Earth Engine to process petabytes of satellite imagery and environmental data. In 2025, Earth Engine’s machine learning models enabled real-time monitoring of deforestation, urban expansion, and water resource changes at unprecedented spatial and temporal resolutions. These insights have informed policy decisions and conservation strategies in collaboration with governmental and non-governmental organizations worldwide.
Looking ahead, the outlook for AI-driven scientific data analysis platforms is robust. Major technology providers are investing in more interpretable and domain-specific AI models, while partnerships with research institutions are expanding. The integration of generative AI, such as large language models, is expected to further enhance hypothesis generation and experimental design. As data volumes continue to grow, these platforms will play an increasingly central role in scientific discovery, enabling breakthroughs that were previously unattainable through traditional methods.
Challenges, Barriers, and Opportunities for Stakeholders
AI-driven scientific data analysis platforms are rapidly transforming research across disciplines, but their adoption in 2025 faces a complex landscape of challenges, barriers, and emerging opportunities for stakeholders. One of the foremost challenges is data interoperability. Scientific data is often siloed in proprietary formats or legacy systems, making integration with AI platforms difficult. Efforts by organizations such as IBM and Microsoft to develop open standards and cloud-based data lakes are helping, but harmonizing diverse datasets remains a technical and organizational hurdle.
Another significant barrier is data privacy and security. As AI platforms increasingly handle sensitive biomedical, environmental, and industrial data, ensuring compliance with evolving regulations (such as GDPR and HIPAA) is critical. Companies like Google and Oracle are investing in secure cloud infrastructures and federated learning approaches to address these concerns, but balancing accessibility with confidentiality continues to be a moving target.
A further challenge is the shortage of domain-specific AI expertise. While general AI tools are proliferating, scientific research often requires highly specialized models and workflows. This skills gap is being addressed through partnerships between technology providers and research institutions. For example, IBM collaborates with universities and national labs to co-develop tailored AI solutions for fields like materials science and genomics. However, scaling such collaborations to meet global demand remains a work in progress.
On the opportunity side, the integration of AI with high-throughput experimental platforms is enabling unprecedented acceleration of discovery. Companies such as Thermo Fisher Scientific and Siemens are embedding AI-driven analytics into laboratory automation and industrial R&D workflows, allowing for real-time data analysis and adaptive experimentation. This not only boosts productivity but also opens new business models for data-driven research services.
Looking ahead, stakeholders can expect further democratization of AI tools, with user-friendly interfaces and low-code platforms lowering the barrier to entry for non-experts. Initiatives by Microsoft and IBM to provide cloud-based AI workbenches are likely to expand access to advanced analytics. At the same time, the push for open science and FAIR (Findable, Accessible, Interoperable, Reusable) data principles is creating opportunities for cross-sector collaboration and innovation. As these trends converge, stakeholders who invest in robust data governance, interdisciplinary partnerships, and continuous upskilling will be best positioned to capitalize on the transformative potential of AI-driven scientific data analysis platforms in the coming years.
Future Outlook: Emerging Trends and Strategic Recommendations
AI-driven scientific data analysis platforms are poised for significant transformation in 2025 and the coming years, driven by rapid advances in machine learning, cloud computing, and domain-specific AI models. The integration of generative AI and large language models (LLMs) is expected to further automate and accelerate scientific discovery, enabling researchers to process and interpret complex datasets with unprecedented speed and accuracy.
A key trend is the increasing adoption of cloud-based AI platforms by leading technology providers. Google Cloud and Microsoft Azure are expanding their AI and machine learning services tailored for scientific research, offering scalable infrastructure and pre-built models for genomics, materials science, and climate modeling. Amazon Web Services (AWS) continues to enhance its AI/ML stack, supporting research institutions with tools for data ingestion, annotation, and advanced analytics. These platforms are increasingly integrating with open-source frameworks and domain-specific libraries, fostering collaboration and reproducibility in scientific workflows.
Specialized AI platforms are also gaining traction. IBM is advancing its AI-powered automation for scientific data analysis, leveraging its Watson platform to support hypothesis generation and experimental design in life sciences and chemistry. Thermo Fisher Scientific and PerkinElmer are embedding AI into laboratory informatics solutions, enabling automated image analysis, anomaly detection, and predictive modeling in drug discovery and diagnostics. In the field of materials science, Schrödinger and Ansys are integrating AI-driven simulation and data analysis tools to accelerate the design of new compounds and materials.
Looking ahead, the convergence of AI with edge computing and the Internet of Things (IoT) is expected to enable real-time data analysis at the source, particularly in environmental monitoring, remote sensing, and laboratory automation. The emergence of foundation models trained on scientific literature and experimental data—such as those being developed by DeepMind and Anthropic—will further enhance the ability of platforms to generate insights, suggest experiments, and even draft scientific reports.
Strategically, organizations should prioritize interoperability, data governance, and ethical AI practices as they adopt these platforms. Investing in workforce upskilling and fostering partnerships with technology providers will be critical to fully leverage the capabilities of AI-driven scientific data analysis. As regulatory frameworks evolve, compliance with data privacy and transparency standards will become increasingly important for both platform providers and end users.
Sources & References
- IBM
- Microsoft
- Elsevier
- Thermo Fisher Scientific
- PerkinElmer
- Sartorius
- Siemens
- Amazon
- Oracle
- DeepMind
- Benchling
- Illumina
- Esri
- CERN
- Bruker
- Google Cloud
- Amazon Web Services
- Schrödinger
- Anthropic