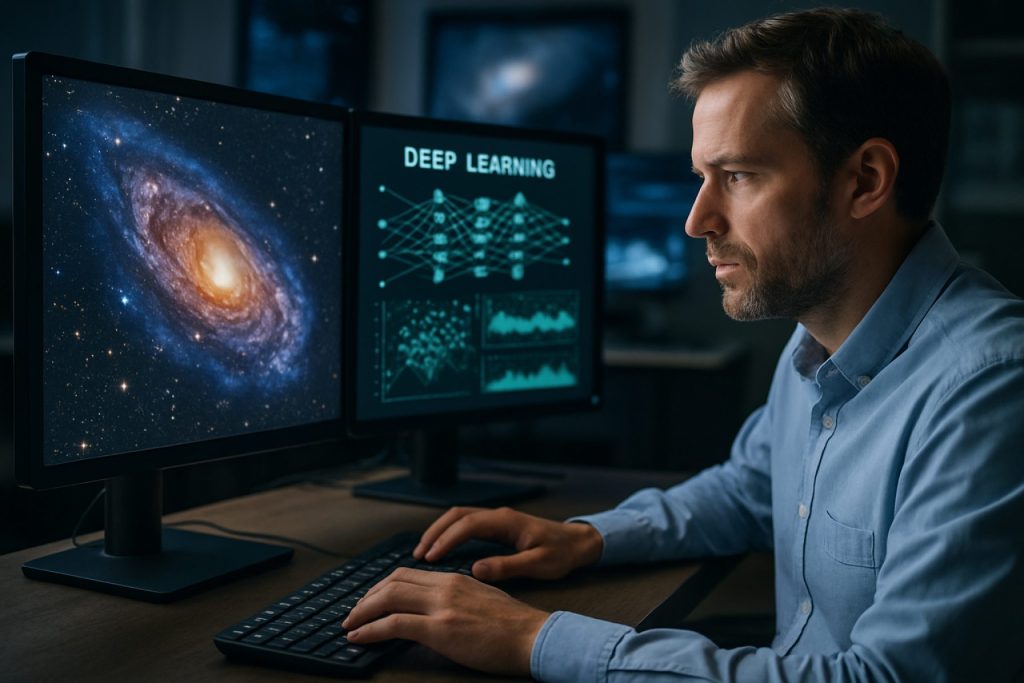
How Deep Learning is Transforming Astronomical Data Classification in 2025: Unveiling Breakthroughs, Market Growth, and the Future of Space Data Analysis
- Executive Summary: 2025 Market Landscape and Key Drivers
- Deep Learning Technologies Shaping Astronomical Data Classification
- Major Industry Players and Strategic Collaborations
- Current Applications: From Exoplanet Detection to Galaxy Morphology
- Market Size, Segmentation, and 2025–2030 Growth Forecasts (CAGR: 18–22%)
- Challenges: Data Volume, Labeling, and Model Interpretability
- Emerging Trends: Self-Supervised Learning and Real-Time Analysis
- Regulatory, Ethical, and Data Governance Considerations
- Case Studies: Leading Projects and Institutional Initiatives (e.g., nasa.gov, esa.int, eso.org)
- Future Outlook: Opportunities, Investment Hotspots, and Next-Gen Innovations
- Sources & References
Executive Summary: 2025 Market Landscape and Key Drivers
The market landscape for deep learning in astronomical data classification is poised for significant growth in 2025, driven by the exponential increase in data volumes from next-generation telescopes and space missions. As observatories such as the Vera C. Rubin Observatory and the European Space Agency’s Euclid mission commence full-scale operations, the astronomical community faces unprecedented data challenges. These facilities are expected to generate petabytes of imaging and spectroscopic data annually, necessitating advanced machine learning solutions for efficient and accurate classification of celestial objects and phenomena.
Deep learning, particularly convolutional neural networks (CNNs) and transformer-based architectures, has emerged as a critical technology for automating the identification and categorization of stars, galaxies, supernovae, and transient events. In 2025, collaborations between leading research institutions and technology companies are accelerating the deployment of scalable AI pipelines. For example, IBM is actively partnering with astronomical research centers to optimize deep learning workflows on high-performance computing infrastructure, while NVIDIA continues to provide specialized GPU hardware and software libraries tailored for large-scale astronomical data analysis.
Key drivers shaping the market include the need for real-time event detection, improved classification accuracy, and the ability to handle multi-modal data streams from diverse instruments. The integration of deep learning with cloud-based platforms is also enabling broader access to computational resources, as seen in initiatives supported by Google Cloud and Microsoft Azure. These platforms are facilitating collaborative research and democratizing access to advanced AI tools for astronomers worldwide.
Looking ahead, the outlook for deep learning in astronomical data classification remains robust. The anticipated launch of additional space telescopes, such as the NASA Nancy Grace Roman Space Telescope, will further amplify data volumes and complexity, reinforcing the demand for automated classification systems. Industry stakeholders are also investing in explainable AI techniques to enhance the interpretability and trustworthiness of deep learning models, a critical factor for scientific discovery and validation.
In summary, 2025 marks a pivotal year for the adoption of deep learning in astronomy, with market growth underpinned by technological innovation, expanding data sources, and strategic partnerships between academia and industry. The sector is expected to witness continued advancements in model performance, scalability, and accessibility, positioning deep learning as an indispensable tool for the next era of astronomical exploration.
Deep Learning Technologies Shaping Astronomical Data Classification
The rapid expansion of astronomical data, driven by next-generation telescopes and sky surveys, is propelling deep learning to the forefront of astronomical data classification in 2025. Modern observatories such as the Vera C. Rubin Observatory, with its Legacy Survey of Space and Time (LSST), are generating petabyte-scale datasets, necessitating automated, scalable, and highly accurate classification systems. Deep learning, particularly convolutional neural networks (CNNs) and transformer-based architectures, is now integral to processing and interpreting this deluge of data.
In 2025, deep learning models are being deployed to classify galaxies, stars, supernovae, and transient events with unprecedented speed and accuracy. The National Aeronautics and Space Administration (NASA) and the European Space Agency (ESA) are leveraging deep learning for missions such as Euclid and the James Webb Space Telescope, where automated classification accelerates the identification of rare or novel phenomena. These agencies are also investing in collaborative platforms and open-source tools to facilitate the sharing and benchmarking of deep learning models across the global astronomy community.
Key industry players are contributing advanced hardware and software solutions to support these efforts. NVIDIA Corporation is providing high-performance GPUs and AI frameworks optimized for large-scale astronomical data processing, enabling real-time inference and training of complex models. IBM and Intel Corporation are also active, offering AI-accelerated computing platforms and cloud-based services tailored for scientific workloads, including astronomy.
Recent breakthroughs include the integration of self-supervised and transfer learning techniques, which allow models to learn from limited labeled data and adapt to new types of astronomical objects. The adoption of explainable AI (XAI) is another trend, as researchers seek to interpret model decisions and ensure scientific validity. Open datasets and competitions, such as those hosted by Kaggle in partnership with leading observatories, are fostering innovation and benchmarking in the field.
Looking ahead, the outlook for deep learning in astronomical data classification is robust. The anticipated launch of new space- and ground-based observatories, such as the Square Kilometre Array (SKA), will further amplify data volumes and complexity. This will drive continued investment in scalable AI infrastructure, federated learning for cross-institutional collaboration, and the development of domain-specific neural architectures. As deep learning matures, it is expected to not only automate routine classification tasks but also enable the discovery of previously unknown astrophysical phenomena, fundamentally advancing our understanding of the universe.
Major Industry Players and Strategic Collaborations
The landscape of deep learning for astronomical data classification in 2025 is shaped by a dynamic interplay between major technology companies, space agencies, and academic consortia. These entities are leveraging advanced machine learning architectures to address the exponential growth in data generated by next-generation telescopes and space missions.
A central player is NASA, which continues to spearhead collaborations through its Astrophysics Data System and partnerships with leading universities. NASA’s ongoing missions, such as the James Webb Space Telescope (JWST) and the upcoming Nancy Grace Roman Space Telescope, are producing petabyte-scale datasets. To manage and classify this data, NASA has intensified its collaboration with cloud and AI leaders, notably Microsoft and Google, integrating their cloud-based AI platforms for scalable deep learning workflows.
The European Space Agency (ESA) is another major force, particularly through its Gaia mission, which is cataloging over a billion stars. ESA’s partnerships with European research institutes and technology providers are focused on developing deep learning models for star classification, anomaly detection, and transient event identification. ESA’s open data policies have also fostered collaborations with AI startups and academic groups across Europe.
In the private sector, IBM has established itself as a key technology provider, offering AI-powered platforms tailored for astronomical data analysis. IBM’s collaborations with observatories and research consortia are enabling the deployment of deep neural networks for real-time event detection and classification. Similarly, NVIDIA is providing the GPU infrastructure and software libraries (such as CUDA and cuDNN) that underpin many deep learning pipelines in astronomy, supporting both academic and commercial initiatives.
Strategic collaborations are also emerging between observatories and technology companies. The Vera C. Rubin Observatory, set to begin full operations in 2025, is working closely with cloud providers and AI specialists to process its unprecedented data streams. Partnerships with Amazon (via AWS) and Google are central to building scalable, automated classification systems for the observatory’s Legacy Survey of Space and Time (LSST).
Looking ahead, the next few years are expected to see deeper integration of AI research from leading universities with the infrastructure and expertise of major tech companies. This convergence is likely to accelerate the development of robust, interpretable deep learning models for astronomical data, driving new discoveries and operational efficiencies across the sector.
Current Applications: From Exoplanet Detection to Galaxy Morphology
Deep learning has rapidly become a cornerstone technology in the classification of astronomical data, with applications spanning from exoplanet detection to the morphological analysis of galaxies. As of 2025, the astronomical community is leveraging increasingly sophisticated neural network architectures to process the vast and complex datasets generated by modern observatories and space missions.
One of the most prominent applications is in the detection and classification of exoplanets. Missions such as NASA’s Transiting Exoplanet Survey Satellite (TESS) and the European Space Agency’s (ESA) CHEOPS are generating terabytes of photometric data, which deep learning models are now routinely used to analyze. These models excel at identifying the subtle, periodic dips in stellar brightness that indicate the presence of exoplanets, outperforming traditional algorithms in both speed and accuracy. NASA has publicly released several deep learning-based tools and datasets to support this work, fostering a collaborative environment for further advancements (NASA).
In the realm of galaxy morphology, deep convolutional neural networks (CNNs) are being deployed to classify millions of galaxies imaged by surveys such as the Sloan Digital Sky Survey (SDSS) and the upcoming Vera C. Rubin Observatory’s Legacy Survey of Space and Time (LSST). These models can distinguish between spiral, elliptical, and irregular galaxies, and even identify rare or previously unclassified morphologies. The LSST, set to begin full operations in the coming years, is expected to produce 20 terabytes of data per night, making automated, deep learning-driven classification essential (Vera C. Rubin Observatory).
Beyond these headline applications, deep learning is also being used for transient event detection (such as supernovae and gamma-ray bursts), star-galaxy separation, and the identification of gravitational lenses. The European Southern Observatory (ESO) and other major facilities are integrating deep learning pipelines into their data processing workflows to handle the increasing data volumes and complexity (European Southern Observatory).
Looking ahead, the next few years will see the deployment of even larger and more sensitive instruments, such as the Square Kilometre Array (SKA), which will further amplify the need for scalable, robust deep learning solutions. Industry leaders in cloud computing and AI hardware, including NVIDIA and IBM, are collaborating with research institutions to optimize deep learning frameworks for astronomical workloads. These partnerships are expected to accelerate the pace of discovery and enable real-time classification of astronomical phenomena at unprecedented scales.
Market Size, Segmentation, and 2025–2030 Growth Forecasts (CAGR: 18–22%)
The market for deep learning applications in astronomical data classification is experiencing robust growth, driven by the exponential increase in data volumes from next-generation telescopes and space missions. As of 2025, the global market size for deep learning in astronomy is estimated to be in the low hundreds of millions USD, with projections indicating a compound annual growth rate (CAGR) between 18% and 22% through 2030. This expansion is fueled by the need to automate the analysis of petabyte-scale datasets generated by observatories such as the Vera C. Rubin Observatory, the Square Kilometre Array (SKA), and the James Webb Space Telescope (JWST).
Market segmentation reveals several key application areas:
- Object Detection and Classification: Deep learning models are increasingly used to identify and classify celestial objects—such as galaxies, stars, exoplanets, and transient phenomena—within massive imaging datasets. This segment is expected to dominate market share, as projects like the Rubin Observatory’s Legacy Survey of Space and Time (LSST) generate tens of terabytes of data nightly.
- Anomaly and Event Detection: Automated detection of rare or unexpected astronomical events (e.g., supernovae, gravitational lensing) is a rapidly growing segment, with deep learning enabling real-time alerts and follow-up observations.
- Spectral Analysis: Deep learning is being applied to classify and interpret spectral data, aiding in the identification of chemical compositions and physical properties of celestial bodies.
- Data Reduction and Noise Filtering: Advanced neural networks are used to preprocess raw data, improving signal-to-noise ratios and enabling more accurate downstream analysis.
Key players in this market include both technology providers and research organizations. IBM and NVIDIA are prominent for their AI hardware and software platforms, which are widely adopted in astronomical research. Intel is also active, providing high-performance computing solutions for large-scale data processing. On the institutional side, organizations such as the European Southern Observatory and the NASA are investing in deep learning infrastructure and collaborative projects to accelerate scientific discovery.
Looking ahead to 2030, the market is expected to benefit from continued advances in AI model architectures, edge computing, and cloud-based data sharing. The integration of deep learning with real-time data streams from new observatories will further expand the addressable market, while partnerships between technology companies and astronomical institutions are likely to drive innovation and adoption. As a result, deep learning is poised to become an indispensable tool for astronomical data classification, underpinning the next wave of discoveries in the field.
Challenges: Data Volume, Labeling, and Model Interpretability
The application of deep learning to astronomical data classification is rapidly advancing, but several critical challenges persist as the field enters 2025. The foremost issue is the sheer volume of data generated by modern and upcoming astronomical surveys. Projects like the Vera C. Rubin Observatory’s Legacy Survey of Space and Time (LSST) are expected to produce tens of terabytes of imaging data per night, resulting in petabyte-scale datasets over their operational lifetimes. This data deluge strains storage, transfer, and processing infrastructure, necessitating scalable solutions for both data management and real-time analysis. Organizations such as Vera C. Rubin Observatory and European Space Agency are actively developing distributed computing frameworks and cloud-based pipelines to address these bottlenecks.
Another significant challenge is the scarcity of high-quality labeled data. Deep learning models require large, accurately annotated datasets for supervised training, but manual labeling of astronomical sources—such as galaxies, stars, and transients—is labor-intensive and often infeasible at scale. Citizen science initiatives, like those coordinated by Zooniverse, have helped generate labeled datasets through public participation, but the complexity and subtlety of many astronomical phenomena limit the effectiveness of this approach. As a result, semi-supervised, self-supervised, and active learning methods are gaining traction, allowing models to leverage vast amounts of unlabeled data and limited expert annotations. The development of synthetic data, using simulations from organizations like NASA and European Space Agency, is also being explored to augment training sets and improve model robustness.
Model interpretability remains a pressing concern, especially as deep learning systems are increasingly used to identify rare or novel astronomical events. The “black box” nature of many neural networks makes it difficult for astronomers to understand the reasoning behind classifications, which is problematic for scientific discovery and validation. Efforts are underway to integrate explainable AI (XAI) techniques, such as saliency mapping and feature attribution, into astronomical pipelines. Organizations like NASA and European Southern Observatory are supporting research into interpretable models that can provide insights into both the data and the decision-making process of the algorithms.
Looking ahead, the astronomical community is expected to prioritize the development of scalable, interpretable, and data-efficient deep learning methods. Collaboration between observatories, space agencies, and the broader AI research community will be essential to overcome these challenges and fully realize the potential of deep learning for astronomical discovery in the coming years.
Emerging Trends: Self-Supervised Learning and Real-Time Analysis
The field of astronomical data classification is undergoing rapid transformation, driven by the integration of deep learning techniques—particularly self-supervised learning and real-time analysis. As astronomical surveys and observatories generate petabytes of data annually, the need for scalable, efficient, and accurate classification methods has never been greater. In 2025 and the coming years, several key trends are shaping the landscape.
Self-supervised learning, which leverages unlabeled data to pre-train models before fine-tuning on smaller labeled datasets, is gaining traction in astronomy. This approach is especially valuable given the scarcity of labeled astronomical data and the abundance of raw observations. Major observatories, such as the Vera C. Rubin Observatory, are preparing for the Legacy Survey of Space and Time (LSST), which will produce tens of terabytes of imaging data nightly. The LSST’s data management system is being designed to support advanced machine learning workflows, including self-supervised and semi-supervised approaches, to enable rapid and robust classification of transient events and celestial objects (Vera C. Rubin Observatory).
Real-time analysis is another emerging trend, necessitated by the sheer volume and velocity of incoming data streams. The Square Kilometre Array (SKA), set to become the world’s largest radio telescope, is expected to generate exabytes of data per year. To manage this, the SKA is investing in high-performance computing infrastructure and developing deep learning pipelines capable of real-time event detection and classification (Square Kilometre Array Observatory). These pipelines are designed to identify phenomena such as fast radio bursts, gravitational wave counterparts, and supernovae within seconds of detection, enabling rapid follow-up observations.
Industry leaders in AI hardware, such as NVIDIA, are collaborating with astronomical institutions to optimize GPU-accelerated deep learning frameworks for large-scale data processing. Their platforms are being integrated into observatory data centers to facilitate both training and inference of complex neural networks on astronomical datasets.
Looking ahead, the convergence of self-supervised learning and real-time analysis is expected to unlock new scientific discoveries. Automated classification systems will not only improve the efficiency of data processing but also enhance the ability to detect rare or previously unknown astronomical events. As observatories like the Rubin Observatory and SKA come online, the astronomical community anticipates a surge in the adoption of deep learning-driven workflows, setting the stage for a new era of data-driven discovery.
Regulatory, Ethical, and Data Governance Considerations
The rapid adoption of deep learning for astronomical data classification is prompting significant attention to regulatory, ethical, and data governance considerations as we move through 2025 and into the coming years. The sheer scale and complexity of astronomical datasets—generated by observatories, satellites, and large-scale surveys—necessitate robust frameworks to ensure responsible data use, transparency, and reproducibility.
A key regulatory concern is the stewardship of data collected by publicly funded observatories and space agencies. Organizations such as the National Aeronautics and Space Administration (NASA) and the European Space Agency (ESA) have established open data policies, mandating that most astronomical data be made freely available to the global research community. These policies are evolving to address the unique challenges posed by deep learning, such as the need for standardized metadata, data provenance tracking, and the reproducibility of machine learning models trained on these datasets.
Ethical considerations are also at the forefront. As deep learning models become more autonomous in classifying celestial objects and detecting transient events, there is a growing emphasis on algorithmic transparency and explainability. The International Astronomical Union (IAU) and other scientific bodies are encouraging the development of guidelines for the responsible use of artificial intelligence in astronomy, including the documentation of model architectures, training data, and performance metrics. This is particularly important for ensuring that scientific discoveries remain verifiable and that biases in training data do not propagate into published results.
Data governance frameworks are being strengthened to address issues of data integrity, security, and long-term preservation. Initiatives like the Vera C. Rubin Observatory (formerly LSST) are setting new standards for data management, with comprehensive policies for data access, user authentication, and audit trails. These frameworks are designed to support collaborative research while protecting sensitive information, such as proprietary data from early-access surveys or embargoed discoveries.
Looking ahead, the integration of deep learning into astronomical workflows is expected to drive further harmonization of data standards and governance practices across international collaborations. The adoption of FAIR (Findable, Accessible, Interoperable, Reusable) data principles is gaining momentum, supported by organizations like the European Southern Observatory (ESO). As regulatory and ethical frameworks mature, they will play a crucial role in ensuring that deep learning accelerates scientific discovery in astronomy while upholding the highest standards of integrity and openness.
Case Studies: Leading Projects and Institutional Initiatives (e.g., nasa.gov, esa.int, eso.org)
In recent years, deep learning has become a cornerstone technology for astronomical data classification, with major space agencies and observatories spearheading innovative projects. As astronomical surveys generate petabytes of data annually, the need for automated, accurate classification systems has never been greater. Several leading institutions have launched initiatives leveraging deep learning to address these challenges, with significant developments expected through 2025 and beyond.
The National Aeronautics and Space Administration (NASA) has been at the forefront of integrating deep learning into its data analysis pipelines. NASA’s Frontier Development Lab (FDL) has collaborated with industry partners to develop neural network models for classifying exoplanets, supernovae, and other transient phenomena from missions such as TESS and Kepler. These models have demonstrated the ability to outperform traditional algorithms in both speed and accuracy, enabling rapid identification of rare astronomical events. In 2025, NASA is expected to expand these efforts as new missions like the Nancy Grace Roman Space Telescope come online, further increasing data volumes and the demand for scalable AI solutions.
The European Space Agency (ESA) has similarly prioritized deep learning for its flagship missions. The Gaia mission, which is mapping over a billion stars, has adopted convolutional neural networks (CNNs) and recurrent neural networks (RNNs) to classify variable stars and detect anomalies in stellar data. ESA’s Euclid mission, launched in 2023, is generating vast imaging datasets to study dark energy and dark matter. Deep learning models are being developed to classify galaxy morphologies and identify gravitational lensing events, with results expected to be published and operationalized through 2025.
Ground-based observatories are also embracing deep learning. The European Southern Observatory (ESO) has implemented machine learning pipelines for the Very Large Telescope (VLT) and is preparing for the data deluge from the upcoming Extremely Large Telescope (ELT). These systems use deep neural networks to classify spectra, identify transient objects, and filter out false positives in real time. ESO’s collaborations with academic and industry partners are expected to yield new open-source tools and frameworks for the broader astronomical community.
Looking ahead, these institutional initiatives are setting the stage for a new era of astronomical discovery. As deep learning models become more sophisticated and datasets grow, the synergy between AI and astronomy will likely accelerate, enabling the detection and classification of phenomena previously beyond human reach. The next few years will see increased cross-institutional collaboration, open data sharing, and the integration of AI-driven classification into mission-critical workflows, fundamentally transforming how astronomers explore the universe.
Future Outlook: Opportunities, Investment Hotspots, and Next-Gen Innovations
The future of deep learning for astronomical data classification is poised for significant advancements, driven by the exponential growth in data volumes from next-generation telescopes and the increasing sophistication of machine learning algorithms. As we move through 2025 and into the latter half of the decade, several key opportunities and investment hotspots are emerging, alongside transformative innovations that promise to reshape the landscape of astronomical research.
One of the most prominent drivers is the deployment of large-scale survey instruments such as the Vera C. Rubin Observatory, which is expected to generate tens of terabytes of imaging data nightly. The observatory’s Legacy Survey of Space and Time (LSST) will require robust, scalable deep learning models to classify transient events, galaxies, and other celestial objects in real time. This creates a fertile ground for investment in AI infrastructure, cloud-based data processing, and automated classification pipelines. Organizations like National Science Foundation and European Southern Observatory are actively funding projects that integrate deep learning into astronomical workflows, recognizing the necessity of AI for handling data at this unprecedented scale.
Commercial technology providers are also entering the field, offering specialized hardware and software solutions tailored for scientific deep learning. Companies such as NVIDIA are collaborating with research institutions to optimize GPU architectures for astronomical workloads, while cloud service providers like Microsoft and Amazon are expanding their AI and data analytics platforms to support large-scale astronomical data processing. These partnerships are expected to accelerate the adoption of deep learning in astronomy, lowering barriers for research groups worldwide.
Looking ahead, next-generation innovations are likely to focus on self-supervised and unsupervised learning techniques, which can leverage vast amounts of unlabeled data to discover novel astrophysical phenomena. The integration of multimodal data—combining optical, radio, and gravitational wave observations—will further enhance classification accuracy and enable new scientific discoveries. Additionally, explainable AI is gaining traction, with researchers and organizations such as European Space Agency prioritizing transparency and interpretability in deep learning models to foster trust and facilitate scientific validation.
In summary, the coming years will see deep learning become an indispensable tool for astronomical data classification, with substantial opportunities for investment in AI infrastructure, cloud computing, and algorithmic innovation. As data volumes continue to surge, the synergy between academia, industry, and government agencies will be crucial in unlocking the full potential of AI-driven astronomy.
Sources & References
- IBM
- NVIDIA
- Google Cloud
- National Aeronautics and Space Administration (NASA)
- European Space Agency (ESA)
- Microsoft
- Amazon
- Vera C. Rubin Observatory
- European Southern Observatory
- Zooniverse
- Square Kilometre Array Observatory
- National Science Foundation