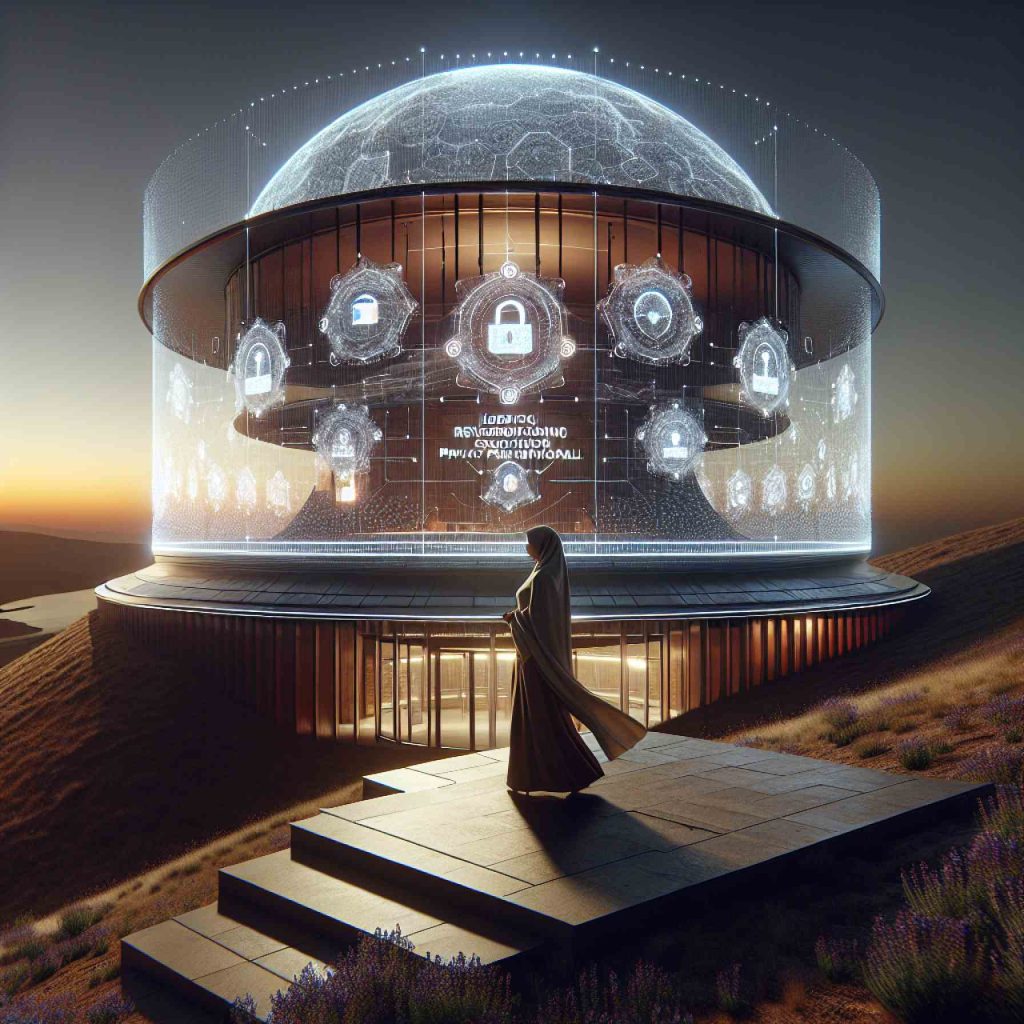
- Nihar Malali, Principal Solutions Architect at National Life Group, is celebrated for his pioneering work on privacy-focused AI technologies.
- His research on federated learning allows AI models to be trained across decentralized devices, enhancing privacy and maintaining efficiency.
- The study features a Hybrid Convolutional Neural Network model achieving a 93.78% accuracy rate on the MNIST dataset, outperforming traditional models.
- Malali’s innovations answer the call for privacy-respecting technologies amidst tightening global regulatory frameworks.
- The research provides practical applications for industries like healthcare and finance, promoting the development of ethical AI systems.
- Malali advocates for “Glass-Box AI,” ensuring systems are both efficient and explainable, aligning with ethical technological standards.
- His work bridges academia and industry, offering a guide for integrating innovation with public trust and ethical considerations.
As the digital landscape evolves at a breakneck pace, data privacy emerges as both a challenge and an opportunity for innovation. At the heart of this intersection is Nihar Malali, the Principal Solutions Architect at National Life Group. Recognized for his pioneering efforts, Malali recently received the “Best Paper Presenter” award at the IEEE International Conference in April 2025 for his work on privacy-focused AI technologies.
Imagine a world where your personal data fuels technological advancement without compromising your privacy—a world increasingly possible due to federated learning. Malali’s acclaimed study delves into this technology, showcasing a revolutionary method that trains machine learning models across decentralized devices. This approach allows the powerful capabilities of AI to flourish while significantly reducing privacy risks.
His research features the Hybrid Convolutional Neural Networks (CNN) model on the foundational MNIST dataset, boasting a remarkable accuracy rate of 93.78%. This figure not only surpasses traditional models such as Multilayer Perceptron and Recurrent Neural Networks but sets a new standard for privacy-compliant accuracy.
But why is this important today? As global regulatory frameworks tighten around data use, there exists a pressing need for innovation that respects privacy. Malali’s federated learning framework answers this call, allowing industries—from healthcare to finance—to enhance AI’s predictive accuracy without the perils of data breaches.
His work doesn’t just remain in academic confines; it offers real-world utility. This cutting-edge research serves as a blueprint for organizations keen to adopt AI responsibly, emphasizing privacy without sacrificing performance. Imagine hospitals jointly developing life-saving predictive models or financial institutions refining fraud detection systems without sharing confidential data—an ethical AI future beckoning.
Malali’s commitment to transparency in AI is underscored by his focus on “Glass-Box AI”—systems that provide explainability alongside efficiency. This philosophy ensures AI remains an ally rather than a master, maintaining accountability and interpretability in a world demanding ethical technological development.
This recent accolade places Malali among the elite of technology thinkers who bridge academia and industry. His work is shaping a landscape where the pursuit of innovation aligns harmoniously with ethical standards and public trust. As organizations navigate the labyrinth of AI and regulatory compliance, Malali’s insights offer an invaluable guide to a future where technology serves humanity with respect and dignity. His guidance serves as a beacon for practitioners, ensuring that the evolution of AI accompanies and enhances human values.
Revolutionizing AI: How Federated Learning Balances Innovation with Data Privacy
The Promise of Federated Learning in Data Privacy
Federated learning is at the forefront of transforming the digital landscape by allowing machine learning models to leverage data without centralizing it. This decentralized approach is pivotal in mitigating privacy risks, effectively addressing the demands of stringent global regulatory frameworks like GDPR and CCPA.
Key Benefits and Applications
1. Healthcare Innovation, Ethically:
– In healthcare, federated learning facilitates collaborative model development without revealing sensitive patient data between institutions, enabling enhanced diagnostics and treatment plans. For instance, hospitals can collaboratively train AI systems for better clinical decision-making while safeguarding patient information.
2. Enhanced Financial Security:
– Financial institutions can utilize federated learning to improve fraud detection systems by pooling knowledge across organizations without sharing proprietary or sensitive bank data, mitigating cybersecurity threats.
3. Improving AI Explainability with Glass-Box AI:
– Transparent AI systems, or “Glass-Box AI,” ensure that decision-making processes are interpretable, maintaining both efficiency and accountability. Organizations benefit by being able to justify AI-driven decisions, which is particularly crucial in high-stakes fields like finance, healthcare, and law.
How Federated Learning Works
1. Initial Model Training:
– A central model is initially trained on a broad dataset to establish a baseline.
2. Decentralized Updating:
– Each participating device or organization downloads the central model, further training occurs locally with their data.
3. Federated Aggregation:
– Updates from all local models are sent back and aggregated at the central server, refining the model while the actual data remains local.
Limitations and Challenges
– Network Dependency:
– Federated learning heavily relies on fast and stable network connections, potentially problematic in regions with inadequate infrastructure.
– Computational Costs:
– Devices must possess significant computational capacity to efficiently train local models, which could limit accessibility for lower-resource setups.
– Potential Biases:
– If the local data isn’t diverse, the aggregated model might reflect biases inherent in the individual datasets.
Future Trends in AI and Federated Learning
– Growing Adoption in Edge Computing:
– As the Internet of Things (IoT) expands, federated learning will likely play a crucial role in edge computing, enabling smart devices to learn without requiring heavy data transfers.
– Increased Focus on AI Ethics:
– Concepts like Glass-Box AI are expected to become industry standards, especially as AI systems make increasingly complex decisions about human livelihoods.
Actionable Recommendations
1. Evaluate Infrastructure:
– Organizations considering federated learning should assess their network and device capabilities to ensure successful implementation.
2. Diverse Data Access:
– When deploying federated learning, ensure participation from diverse datasets to reduce model bias and improve overall accuracy.
3. Investment in Transparency:
– Adopt transparent AI practices to align with evolving regulatory requirements and build trust with consumers and stakeholders.
Additional Resources
– For more on privacy and AI ethics, visit the IEEE.
– Learn about innovative AI applications in healthcare at World Health Organization.
By embracing federated learning, organizations can lead the way in creating AI systems that protect privacy and enhance capabilities, ensuring technology serves humanity effectively and ethically.