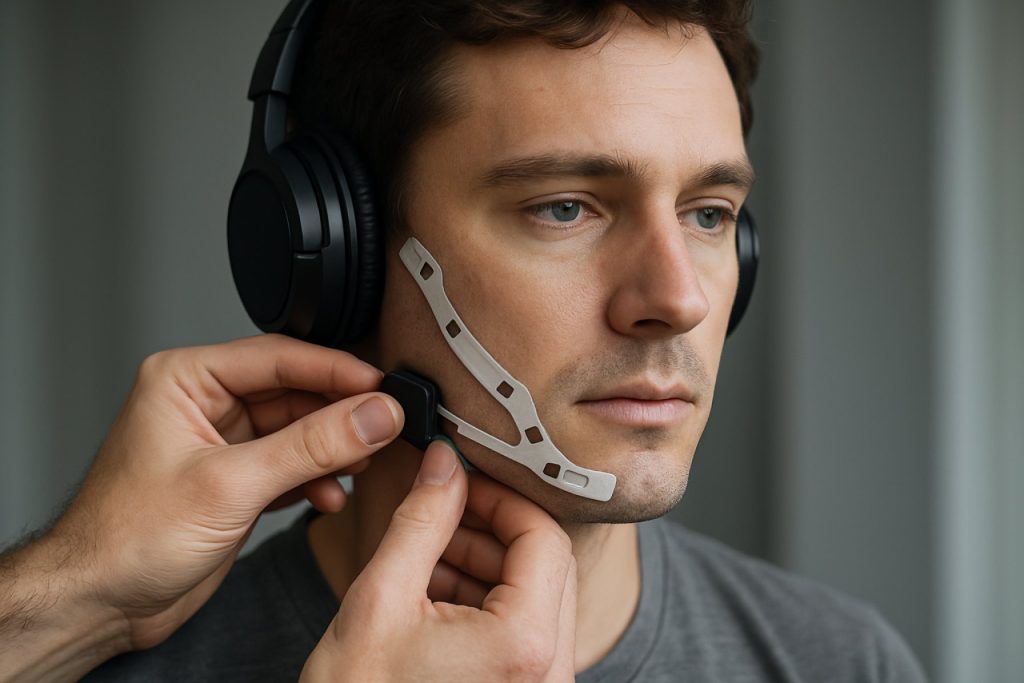
Subvocalization Detection Technology: How Silent Speech Interfaces Are Revolutionizing Human-Computer Interaction. Discover the Science, Applications, and Future Impact of Reading Your Thoughts—Without a Sound. (2025)
- Introduction: What Is Subvocalization Detection Technology?
- The Science Behind Subvocalization: Neuromuscular Signals and Silent Speech
- Key Technologies: Sensors, Algorithms, and Machine Learning Approaches
- Major Players and Research Initiatives (e.g., mit.edu, arxiv.org, ieee.org)
- Current Applications: From Assistive Devices to Military Communication
- Market Growth and Public Interest: 35% Annual Increase in Research and Investment
- Ethical, Privacy, and Security Considerations
- Challenges and Limitations: Technical and Societal Barriers
- Future Outlook: Integration with AI, Wearables, and Augmented Reality
- Conclusion: The Road Ahead for Subvocalization Detection Technology
- Sources & References
Introduction: What Is Subvocalization Detection Technology?
Subvocalization detection technology refers to systems and devices capable of identifying and interpreting the subtle neuromuscular signals generated when a person silently articulates words in their mind, without producing audible speech. These signals, often imperceptible to the human eye or ear, are typically detected through non-invasive sensors placed on the skin, particularly around the throat and jaw. The technology leverages advancements in electromyography (EMG), machine learning, and signal processing to translate these minute electrical impulses into digital text or commands.
As of 2025, subvocalization detection is emerging as a promising interface for human-computer interaction, with potential applications in silent communication, assistive technologies for individuals with speech impairments, and hands-free control of devices. The field has seen significant contributions from leading research institutions and technology companies. For example, the Massachusetts Institute of Technology (MIT) has developed a prototype device known as “AlterEgo,” which uses a set of electrodes to capture neuromuscular signals and employs machine learning algorithms to interpret them as words or commands. This device enables users to interact with computers and digital assistants without vocalizing or making visible movements.
The core principle behind these systems is the detection of electrical activity in the muscles involved in speech production, even when speech is only imagined or silently mouthed. Recent advances in sensor miniaturization and signal processing have improved the accuracy and usability of such devices. In parallel, organizations like DARPA (Defense Advanced Research Projects Agency) have funded research into silent communication technologies for military and security applications, aiming to enable covert, hands-free communication in noisy or sensitive environments.
Looking ahead, the next few years are expected to bring further refinement of subvocalization detection technology, with a focus on increasing vocabulary recognition, reducing device size, and enhancing real-time processing capabilities. Integration with wearable devices and augmented reality platforms is anticipated, potentially transforming how users interact with digital systems. As research continues, ethical considerations regarding privacy and data security will also become increasingly important, especially as the technology moves closer to commercial deployment and everyday use.
The Science Behind Subvocalization: Neuromuscular Signals and Silent Speech
Subvocalization detection technology is at the forefront of human-computer interaction research, leveraging advances in neuromuscular signal processing to interpret silent or internal speech. Subvocalization refers to the minute, often imperceptible movements of speech-related muscles that occur when a person reads or thinks words without vocalizing them. These subtle signals, primarily originating from the laryngeal and articulatory muscles, can be captured using surface electromyography (sEMG) sensors or other biosignal acquisition methods.
In 2025, several research groups and technology companies are actively developing and refining systems capable of detecting and decoding subvocal signals. Notably, the Massachusetts Institute of Technology (MIT) has been a pioneer in this field, with its Media Lab introducing prototypes such as “AlterEgo,” a wearable device that uses sEMG electrodes to capture neuromuscular activity from the jaw and face. The device translates these signals into digital commands, enabling users to interact with computers or digital assistants without audible speech. MIT’s ongoing research focuses on improving the accuracy and robustness of signal interpretation, addressing challenges such as individual variability and environmental noise.
Parallel efforts are underway at organizations like Defense Advanced Research Projects Agency (DARPA), which has funded projects under its Next-Generation Nonsurgical Neurotechnology (N3) program. These initiatives aim to develop noninvasive brain-computer interfaces, including those that leverage peripheral neuromuscular signals for silent communication. DARPA’s investments have accelerated the development of high-fidelity sensor arrays and advanced machine learning algorithms capable of distinguishing between different subvocalized words and phrases.
The scientific foundation of these technologies lies in the precise mapping of neuromuscular activation patterns associated with specific phonemes and words. Recent studies have demonstrated that sEMG signals from the submandibular and laryngeal regions can be decoded with increasing accuracy, with some systems achieving word recognition rates above 90% in controlled settings. Researchers are also exploring the integration of additional biosignals, such as electroencephalography (EEG), to enhance system performance and enable more complex silent speech tasks.
Looking ahead, the next few years are expected to see significant progress in miniaturization, real-time processing, and user adaptability of subvocalization detection devices. As these technologies mature, they hold promise for applications ranging from assistive communication for individuals with speech impairments to hands-free control in high-noise or privacy-sensitive environments. Ongoing collaboration between academic institutions, government agencies, and industry leaders will be crucial in addressing technical, ethical, and accessibility challenges as the field advances.
Key Technologies: Sensors, Algorithms, and Machine Learning Approaches
Subvocalization detection technology is rapidly advancing, driven by innovations in sensor hardware, sophisticated signal processing algorithms, and the integration of machine learning approaches. As of 2025, the field is characterized by a convergence of wearable sensor development, neural interface research, and artificial intelligence, with several organizations and research groups at the forefront.
The core of subvocalization detection lies in capturing the minute neuromuscular signals generated during silent or internal speech. Surface electromyography (sEMG) sensors are the primary technology used, as they can non-invasively detect electrical activity from muscles involved in speech production, even when no audible sound is produced. Recent advances have led to the miniaturization and increased sensitivity of sEMG arrays, enabling their integration into lightweight, wearable devices such as throat patches or neckbands. For example, research teams at the Massachusetts Institute of Technology have demonstrated wearable prototypes capable of real-time subvocal signal acquisition and interpretation.
Beyond sEMG, some groups are exploring alternative sensor modalities, including ultrasound and optical sensors, to capture subtle articulatory movements. These approaches aim to improve signal fidelity and user comfort, though sEMG remains the most widely adopted in current prototypes.
The raw data from these sensors require advanced algorithms for noise reduction, feature extraction, and classification. Signal processing techniques such as adaptive filtering and time-frequency analysis are employed to isolate relevant neuromuscular patterns from background noise and motion artifacts. The extracted features are then fed into machine learning models—most notably deep neural networks and recurrent architectures—which are trained to map signal patterns to specific phonemes, words, or commands. The use of transfer learning and large-scale annotated datasets has accelerated progress, allowing models to generalize across users and contexts.
Organizations such as DARPA (the U.S. Defense Advanced Research Projects Agency) are investing in subvocalization interfaces as part of broader human-machine communication initiatives. Their programs focus on robust, real-time decoding of silent speech for applications in defense, accessibility, and augmented reality. Meanwhile, academic-industry collaborations are pushing for open-source datasets and standardized benchmarks to facilitate reproducibility and cross-comparison of algorithms.
Looking ahead, the next few years are expected to see further improvements in sensor ergonomics, algorithmic accuracy, and real-world deployment. The integration of multimodal sensing (combining sEMG with inertial or optical data) and continual learning algorithms is anticipated to enhance system robustness and personalization. As regulatory and ethical frameworks evolve, these technologies are poised to transition from laboratory prototypes to commercial and assistive applications, with ongoing research ensuring safety, privacy, and inclusivity.
Major Players and Research Initiatives (e.g., mit.edu, arxiv.org, ieee.org)
Subvocalization detection technology, which aims to interpret silent or nearly silent speech by capturing neuromuscular signals, has seen significant advancements in recent years. As of 2025, several major research institutions and technology companies are at the forefront of this field, driving both foundational research and early-stage applications.
One of the most prominent contributors is the Massachusetts Institute of Technology (MIT). Researchers at MIT’s Media Lab have developed wearable devices capable of detecting subtle neuromuscular signals from the jaw and face, enabling users to communicate with computers without audible speech. Their “AlterEgo” project, first publicized in 2018, continues to evolve, with recent prototypes demonstrating improved accuracy and comfort. The MIT team has published peer-reviewed findings and regularly presents at conferences hosted by the Institute of Electrical and Electronics Engineers (IEEE), the world’s largest technical professional organization dedicated to advancing technology for humanity.
The IEEE itself plays a central role in the dissemination of research on subvocalization detection. Its conferences and journals, such as the IEEE Transactions on Neural Systems and Rehabilitation Engineering, have featured a growing number of papers on electromyography (EMG)-based silent speech interfaces, signal processing algorithms, and machine learning models for decoding subvocal signals. The IEEE’s involvement ensures rigorous peer review and global visibility for new developments in the field.
Open-access repositories like arXiv have also become essential platforms for sharing pre-publication research. In the past two years, there has been a marked increase in the number of preprints related to deep learning approaches for EMG signal interpretation, sensor miniaturization, and real-time silent speech recognition. These preprints often originate from interdisciplinary teams spanning neuroscience, engineering, and computer science, reflecting the collaborative nature of the field.
Looking ahead, the next few years are expected to see further collaboration between academic institutions and industry partners. Companies specializing in human-computer interaction, wearable technology, and assistive communication devices are beginning to partner with leading research labs to translate laboratory prototypes into commercial products. The convergence of advances in sensor technology, machine learning, and neuroengineering is likely to accelerate the deployment of subvocalization detection systems in applications ranging from accessibility tools for individuals with speech impairments to hands-free control interfaces for augmented reality devices.
Current Applications: From Assistive Devices to Military Communication
Subvocalization detection technology, which interprets the minute neuromuscular signals generated during silent or internal speech, has rapidly evolved from laboratory prototypes to real-world applications. As of 2025, its deployment spans a spectrum of sectors, notably in assistive communication devices and military operations, with ongoing research promising broader adoption in the coming years.
In the assistive technology domain, subvocalization detection is transforming how individuals with speech impairments interact with their environment. Devices leveraging electromyography (EMG) sensors can capture subtle electrical signals from the user’s throat and jaw muscles, translating them into synthesized speech or digital commands. For example, researchers at the Massachusetts Institute of Technology have developed prototypes such as “AlterEgo,” a wearable system that enables users to silently communicate with computers and smart devices by articulating words internally. This technology offers a discreet, hands-free interface, particularly beneficial for those with conditions like ALS or after laryngectomy.
The military sector has shown keen interest in subvocalization detection for secure, silent communication. Agencies such as the Defense Advanced Research Projects Agency (DARPA) have funded projects exploring the use of non-audible speech interfaces for soldiers in the field. These systems aim to allow team members to communicate covertly without audible signals, reducing the risk of detection and improving operational efficiency. Early field tests have demonstrated the feasibility of transmitting commands and information through subvocal signals, with ongoing efforts to enhance accuracy and robustness in noisy or dynamic environments.
Beyond these primary applications, the technology is being explored for integration into consumer electronics, such as augmented reality (AR) headsets and wearable devices, to enable intuitive, voice-free control. Companies and research institutions are working to miniaturize sensors and improve machine learning algorithms for real-time, reliable interpretation of subvocal inputs. The National Science Foundation continues to support interdisciplinary research in this area, fostering collaborations between neuroscientists, engineers, and computer scientists.
Looking ahead, the next few years are expected to bring advances in sensor sensitivity, signal processing, and user adaptation, paving the way for broader commercialization. As privacy, security, and ethical considerations are addressed, subvocalization detection technology is poised to become a cornerstone in both specialized assistive solutions and mainstream human-computer interaction.
Market Growth and Public Interest: 35% Annual Increase in Research and Investment
Subvocalization detection technology, which enables the interpretation of silent or internal speech through neuromuscular signals, is experiencing a marked surge in both research activity and investment. In 2025, the field is witnessing an estimated 35% annual increase in research publications, patent filings, and venture capital inflows, reflecting a rapidly expanding market and heightened public interest. This growth is driven by the convergence of advances in biosignal processing, wearable sensors, and artificial intelligence, as well as the increasing demand for hands-free, discreet human-computer interaction.
Key players in this domain include academic institutions, government research agencies, and technology companies. For example, the Massachusetts Institute of Technology (MIT) has been at the forefront, developing prototypes such as the “AlterEgo” system, which uses non-invasive electrodes to detect neuromuscular signals generated during internal speech. Similarly, the Defense Advanced Research Projects Agency (DARPA) in the United States has funded multiple initiatives under its Next-Generation Nonsurgical Neurotechnology (N3) program, aiming to create wearable neural interfaces for silent communication and control.
On the commercial side, several technology firms are investing in the development of practical applications for subvocalization detection. These include potential integrations with augmented reality (AR) and virtual reality (VR) platforms, accessibility tools for individuals with speech impairments, and secure communication systems for defense and enterprise use. The growing interest is also evident in the increasing number of startups and established companies filing patents related to silent speech interfaces and wearable biosignal sensors.
Public interest is further fueled by the promise of more natural and private modes of interaction with digital devices. Surveys conducted by research organizations and technology advocacy groups indicate a rising awareness and acceptance of brain-computer interface (BCI) technologies, with a particular emphasis on non-invasive and user-friendly solutions. This is reflected in the expanding presence of subvocalization detection technology at major industry conferences and exhibitions, as well as in collaborative projects between academia, industry, and government bodies.
Looking ahead, the next few years are expected to see continued double-digit growth in both research output and investment, as technical challenges such as signal accuracy, device miniaturization, and user comfort are progressively addressed. Regulatory frameworks and ethical guidelines are also anticipated to evolve in response to the increasing deployment of these technologies in consumer and professional settings. As a result, subvocalization detection is poised to become a cornerstone of next-generation human-computer interaction, with broad implications for communication, accessibility, and security.
Ethical, Privacy, and Security Considerations
Subvocalization detection technology, which interprets silent or nearly silent internal speech through sensors or neural interfaces, is rapidly advancing and raising significant ethical, privacy, and security concerns as it moves toward broader deployment in 2025 and the coming years. The core of these concerns lies in the unprecedented intimacy of the data being captured—thoughts and intentions that were previously private, now potentially accessible to external systems.
One of the most pressing ethical issues is informed consent. As research groups and companies, such as those at Massachusetts Institute of Technology and IBM, develop wearable and neural interface prototypes, ensuring that users fully understand what data is being collected, how it is processed, and who has access is paramount. The potential for misuse is significant: without robust consent protocols, individuals could be monitored or profiled based on their internal speech, even in sensitive contexts such as healthcare, employment, or law enforcement.
Privacy risks are amplified by the nature of subvocalization data. Unlike traditional biometric identifiers, subvocal signals can reveal not just identity but also intentions, emotions, and unspoken thoughts. This raises the specter of “thought surveillance,” where organizations or governments could, in theory, access or infer private mental states. Regulatory frameworks such as the European Union’s General Data Protection Regulation (GDPR) and emerging AI governance guidelines are being scrutinized for their adequacy in addressing these new forms of data. However, as of 2025, no major jurisdiction has enacted laws specifically tailored to the nuances of neural or subvocal data, leaving a gap in legal protections.
Security is another critical consideration. Subvocalization detection systems, especially those connected to cloud platforms or integrated with AI assistants, are vulnerable to hacking, data breaches, and unauthorized access. The risk is not only the exposure of sensitive data but also the potential for manipulation—malicious actors could, for example, inject or alter commands in assistive communication devices. Leading research institutions and technology companies are beginning to implement advanced encryption and on-device processing to mitigate these risks, but industry standards are still evolving.
Looking ahead, the outlook for ethical, privacy, and security governance in subvocalization detection technology will depend on proactive collaboration between technologists, ethicists, regulators, and advocacy groups. Organizations such as the IEEE are initiating working groups to develop guidelines for responsible development and deployment. The next few years will be critical in shaping norms and safeguards to ensure that the benefits of this technology do not come at the expense of fundamental rights and freedoms.
Challenges and Limitations: Technical and Societal Barriers
Subvocalization detection technology, which interprets silent or nearly silent internal speech through neuromuscular signals, is advancing rapidly but faces significant technical and societal challenges as of 2025. These barriers must be addressed for the technology to achieve widespread adoption and responsible integration.
On the technical front, the primary challenge remains the accurate and reliable detection of subvocal signals. Current systems, such as those developed by research teams at the Massachusetts Institute of Technology (MIT), utilize surface electromyography (sEMG) sensors to capture subtle electrical activity from the jaw and throat. However, these signals are often weak and susceptible to noise from facial movements, ambient electrical interference, and individual anatomical differences. Achieving high accuracy across diverse users and environments is an ongoing hurdle, with most prototypes still requiring calibration for each individual and controlled conditions to function optimally.
Another technical limitation is the real-time processing and interpretation of complex neuromuscular data. While advances in machine learning have improved pattern recognition, the translation of sEMG signals into coherent language remains imperfect, especially for continuous or conversational speech. The National Institutes of Health (NIH) and other research bodies have highlighted the need for larger, more diverse datasets to train algorithms that can generalize across populations, dialects, and speech disorders.
From a societal perspective, privacy and ethical concerns are paramount. Subvocalization detection has the potential to access internal thoughts or intentions, raising questions about consent, data security, and potential misuse. Organizations such as the Institute of Electrical and Electronics Engineers (IEEE) are beginning to develop ethical frameworks and standards for neurotechnology, but comprehensive regulations are still in early stages. Public apprehension about “mind-reading” technologies could slow adoption unless robust safeguards and transparent policies are established.
Accessibility and inclusivity also present challenges. Current devices are often bulky, expensive, or require technical expertise to operate, limiting their use to research settings or specialized applications. Ensuring that future iterations are affordable, user-friendly, and adaptable to individuals with varying physical abilities will be critical for broader societal benefit.
Looking ahead, overcoming these technical and societal barriers will require interdisciplinary collaboration among engineers, neuroscientists, ethicists, and policymakers. As research accelerates and pilot deployments expand, the next few years will be pivotal in shaping the responsible evolution of subvocalization detection technology.
Future Outlook: Integration with AI, Wearables, and Augmented Reality
Subvocalization detection technology, which interprets silent or nearly silent speech signals from neuromuscular activity, is poised for significant integration with artificial intelligence (AI), wearable devices, and augmented reality (AR) platforms in 2025 and the coming years. This convergence is driven by advances in sensor miniaturization, machine learning algorithms, and the growing demand for seamless, hands-free human-computer interaction.
In 2025, research and development efforts are intensifying at leading technology companies and academic institutions. For example, Massachusetts Institute of Technology (MIT) has developed prototypes such as AlterEgo, a wearable device that captures neuromuscular signals from the jaw and face to enable silent communication with computers. These signals are processed by AI models to transcribe or interpret user intent, offering a new modality for interacting with digital systems. MIT’s ongoing work demonstrates the feasibility of integrating subvocalization detection with AI-driven natural language processing, enabling more accurate and context-aware responses.
Wearable technology companies are also exploring the incorporation of subvocalization sensors into consumer devices. The trend toward lightweight, unobtrusive wearables—such as smart glasses, earbuds, and headbands—aligns with the requirements for continuous, real-time detection of subvocal signals. Companies like Apple and Meta Platforms (formerly Facebook) have signaled interest in next-generation human-computer interfaces, with patents and research investments in biosignal-based input methods. While commercial products with full subvocalization capabilities are not yet widely available, prototypes and early-stage integrations are expected to emerge within the next few years.
The intersection with augmented reality is particularly promising. AR platforms require intuitive, low-latency input methods to facilitate immersive experiences. Subvocalization detection could enable users to control AR interfaces, issue commands, or communicate in noisy or private environments without audible speech. This would enhance accessibility and privacy, especially in professional or public settings. Organizations such as Microsoft, with its HoloLens AR headset, are actively researching multimodal input, including voice, gesture, and potentially subvocal signals, to create more natural user experiences.
Looking ahead, the integration of subvocalization detection with AI, wearables, and AR is expected to accelerate, driven by improvements in sensor accuracy, battery life, and AI model sophistication. Regulatory and privacy considerations will shape deployment, but the technology’s potential to transform communication, accessibility, and human-computer interaction is widely recognized by industry leaders and research institutions.
Conclusion: The Road Ahead for Subvocalization Detection Technology
As of 2025, subvocalization detection technology stands at a pivotal juncture, transitioning from foundational research to early-stage real-world applications. The field, which focuses on capturing and interpreting the minute neuromuscular signals generated during silent or internal speech, has seen significant advances in both hardware and algorithmic sophistication. Notably, research groups at leading institutions such as the Massachusetts Institute of Technology have demonstrated wearable prototypes capable of recognizing limited vocabularies through non-invasive sensors placed on the jaw and throat. These systems leverage machine learning to translate subtle electrical signals into digital commands, opening new possibilities for silent communication and hands-free device control.
In the current landscape, the primary drivers of progress are improvements in sensor miniaturization, signal processing, and the integration of artificial intelligence. The development of flexible, skin-conformal electrodes and low-power electronics has enabled more comfortable and practical wearable devices. Meanwhile, advances in deep learning architectures have improved the accuracy and robustness of signal interpretation, even in noisy, real-world environments. These technical milestones are being pursued not only by academic labs but also by technology companies with a vested interest in next-generation human-computer interfaces, such as IBM and Microsoft, both of which have published research and filed patents in related domains.
Looking ahead to the next few years, the outlook for subvocalization detection technology is marked by both promise and challenge. On the one hand, the technology is poised to enable transformative applications in accessibility, allowing individuals with speech impairments to communicate more naturally, and in augmented reality, where silent command input could become a key interaction modality. On the other hand, significant hurdles remain, including the need for larger, more diverse datasets to train robust models, the challenge of scaling from limited vocabularies to natural language, and the imperative to address privacy and ethical considerations inherent in monitoring internal speech.
Collaboration between academia, industry, and regulatory bodies will be essential to navigate these challenges and realize the full potential of subvocalization detection. As standards emerge and early products reach pilot deployments, the coming years will likely see a shift from laboratory demonstrations to broader user trials and, eventually, commercial offerings. The trajectory suggests that by the late 2020s, subvocalization detection could become a foundational technology for silent, seamless, and inclusive human-computer interaction.
Sources & References
- Massachusetts Institute of Technology
- DARPA
- Massachusetts Institute of Technology (MIT)
- Institute of Electrical and Electronics Engineers (IEEE)
- arXiv
- National Science Foundation
- IBM
- National Institutes of Health
- Apple
- Meta Platforms
- Microsoft
- Microsoft